Redesigning Instruction & Assessment in the Age of AI
Or, the increasingly critical role of instructional design in the AI world
Prefer to listen rather than read? Here’s NotebookLM’s podcast version of this week’s post (with all of the necessary caveats - note how AI assumes I’m male…)
Hey folks!
This week, I found some time to read a research paper by Charles B. Hodges and Paul A. Kirschner titled, "Innovation of Instructional Design and Assessment in the Age of Generative Artificial Intelligence" which was produced in 2023 and published this month in TechTrends.
As we now well, generative AI tools like ChatGPT have become a focal point in education, sparking intense debates about their potential risks, benefits and overall impact. In their article, Hodges and Kirschner raise many of the big questions which are explored as part of this conversation, including:
Will AI tools will “revolutionise” learning?
Do AI tools undermine academic integrity?
Does AI limit deep cognitive engagement?
These questions are now central to majority of conversations about education and training, and are at the heart of Hodges and Kirschner’s article.
So, what did they find?
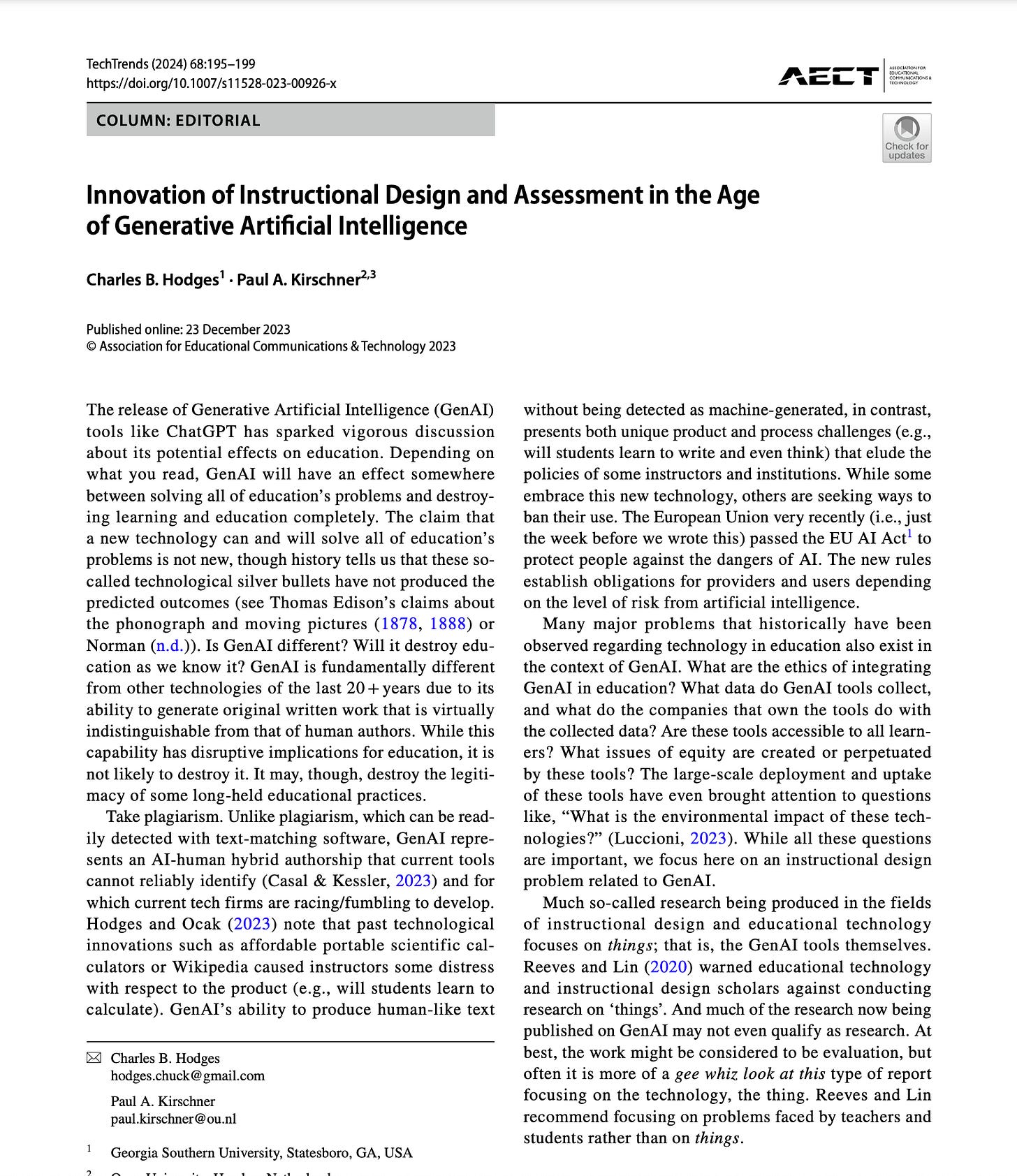
The TLDR is that the authors argue that AI represents a fundamental and unavoidable shift in educational technology. Unlike previous tools like calculators or Wikipedia, AI’s ability to generate human-like text means that traditional forms of teaching and assessment will be (perhaps already are) fundamentally and irreversibly disrupted by AI.
Unlike previous tools like calculators or Wikipedia, AI’s ability to generate human-like text means that traditional forms of teaching and assessment will be (perhaps already are) fundamentally and irreversibly disrupted by AI.
Hodges and Kirschner explore how GenAI raises significant ethical, educational, and practical challenges, including the potential for plagiarism, issues with equity, and the effectiveness of current AI-detection tools.
While some educators remain eager to ban AI tools outright, the authors suggest a different path forward—one where instructional design evolves to meet these new challenges. Instead of focusing on how to integrate or restrict AI, the article calls for a rethink of instructional strategies to ensure students engage in genuine learning.
In this week’s blog, I’d like to build on Hodges and Kirschner’s insights and ask: if education and training are fundamentally disrupted by AI, what does this look like in practice? How will the way that we design, deliver evaluate learning likely change, and what does this look like in practice?
Let’s dive in! 🚀
Summary of the Article: "Innovation of Instructional Design and Assessment in the Age of Generative AI"
Hodges and Kirschner’s article highlights a critical moment in education where the rise of AI tools like ChatGPT has sparked both enthusiasm and concern. The authors acknowledge, in line with my own thinking, that while technological advances often promise to solve educational problems, history shows these promises rarely materialise as expected.
However, AI, they argue, is fundamentally different from previous technologies because it produces original written work that is nearly indistinguishable from that of human authors. This capability disrupts traditional educational practices, particularly established methods of assessment (think: essays).
One of the major concerns discussed in the article is, of course, plagiarism. Unlike traditional plagiarism, which can be detected by text-matching software, Gen AI presents a hybrid form of authorship that makes it difficult to distinguish between human- and AI-generated content. Current AI-detection tools are still in their infancy, deeply unreliable and problematic, raising questions about how to maintain academic integrity in this new landscape.
Beyond plagiarism, Hodges and Kirschner also highlight broader ethical concerns, such as the accessibility of AI tools, the data they collect, and the equity issues they perpetuate. They also touch on the environmental impact of large-scale AI deployment which, as Google’s recent investment in nuclear power plants to power AI shows, is not to be underestimated.
However, the core problem that Hodges and Kirschner identify is how instructional design - i.e. the way we conceive of and design “learning experiences” - must evolve to address these challenges. The authors argue that instead of banning AI or integrating it without a clear pedagogical strategy, educators must rethink the design of instruction and assessment to ensure that genuine learning occurs.
To this end, they propose several strategies, including emphasising the learning process over the final product, incorporating oral assessments, modifying assignments to be more specific and context-dependent, and using AI tools critically rather than passively.
Hodges and Kirschner’s concluding call to action is clear: education must shift its focus from traditional assessments that AI can easily complete to fostering critical thinking, creativity, and deep understanding.
But what would this look like in practice?
Instructional Strategies in the AI Era: a Case Study
While Hodges and Kirschner lay a strong foundation, their suggestions invite further exploration. As AI tools continue to evolve, it’s no longer enough to simply adapt existing instructional designs. We need to rethink the entire approach to instruction and assessment in a world where AI is a ubiquitous tool.
To do this, I hope to expand on and add value to the this research by offering my thoughts on some concrete instructional strategies that promote genuine learning, regardless of whether or not AI is involved.
Let’s start with a case study, specifically the Master’s in Global Diplomacy at the University of London (picked at random from a list of courses which share their syllabus info publicly).
Using this document, I put together a “before and after” comparison of how the teaching, content, and assessment of a course like this might evolve in the AI-world.
Designing & Assessing Learning in the AI Era
Based on Hodges and Kirschner’s work and my own research, I’ve started to develop some key strategies for instructional and assessment design in the AI era. Here’s where I’ve landed so far:
AI-Era Instructional Strategies
1. Inquiry-Based and Problem-Based Learning (PBL)
Why it works: These approaches encourage curiosity and problem-solving, pushing students to explore complex problems that require more than surface-level AI-generated responses.
Practical example: Assign real-world problems, such as environmental or social issues, and require students to research and propose solutions. AI can be used as a research tool, but students must critically evaluate its outputs and apply knowledge from multiple disciplines to develop creative, evidence-based solutions.
2. Collaborative & Social Learning
Why it works: Collaboration fosters communication, problem-solving, and the development of interpersonal skills, areas where AI cannot fully replace human input.
Practical example: Organise group projects where students must work together to solve a problem, using AI tools as assistants for research and brainstorming. Peer review processes can also help deepen understanding, as students critique and provide feedback on each other’s work.
3. Creative and Applied Learning
Why it works: Applied learning tasks encourage students to engage deeply with content and use their knowledge in practical, creative ways.
Practical example: Design projects where students must create a prototype, model, or proposal for a real-world issue. AI can assist in brainstorming and generating ideas, but the final product requires the student’s unique creative application of knowledge.
4. Critical Analysis & Evaluation
Why it works: Critical analysis pushes students to engage deeply with AI-generated content, developing the skills necessary to discern bias, error, and truth.
Practical example: Task students with evaluating AI-generated outputs in relation to specific topics, identifying biases, errors, and gaps in the information. By critically analysing AI content, students build a deeper understanding of the material and of how AI systems work.
5. Focus on Metacognition & Self-Regulation
Why it works: Metacognition helps learners think about their own thinking, ensuring they engage deeply with content rather than relying on AI to do the work.
Practical example: Assign reflective learning journals where students document how they used AI tools in their research, what challenges they faced, and how they overcame them. This promotes self-awareness and ensures students critically engage with AI’s outputs, rather than passively accepting them.
AI-Era Assessment Strategies
1. Process-Oriented Assignments
Why it works: Focusing on the process, rather than just the product, ensures that students engage in authentic learning activities.
Practical example: Require students to submit drafts, outlines, or annotated bibliographies alongside their final submission. By assessing the steps taken during the learning process, educators can see how students are thinking and engaging with the material, not just the polished final product—something that AI can easily generate.
2. Oral & Performance-Based Assessments
Why it works: These assessments are difficult for AI to complete on behalf of the student and require a demonstration of understanding and application.
Practical example: Use oral presentations or real-time demonstrations where students explain their reasoning and the process they followed to complete a task. This ensures students have a deep understanding of the subject and can articulate how they used AI as a tool, not a substitute for their own thinking.
3. Frequent, Low-Stakes Assessments
Why it works: Continuous assessment helps track student progress and ensures they engage with the material throughout the learning process.
Practical example: Incorporate quick quizzes, reflection exercises, or polls during lessons. These assessments can be completed in-class or monitored online, ensuring that students engage with content regularly rather than relying on last-minute AI-generated solutions.
Conclusion: A New Paradigm for Learning in a Post-AI World?
In the AI world, the focus of human learning shifts from the act of remembering and regurgitating to the act of analysing, assessing and creating.
In practice, this means a shift from traditional, individual, essay-based assessments to a more dynamic, collaborative and critically engaged “higher order” learning environment.
By forcing us to rethink how we design and assess “learning”, AI pushes us to imagine, design and deliver a more ambitious version of education and training which delivers dynamic skills & behaviours as well as knowledge gain.
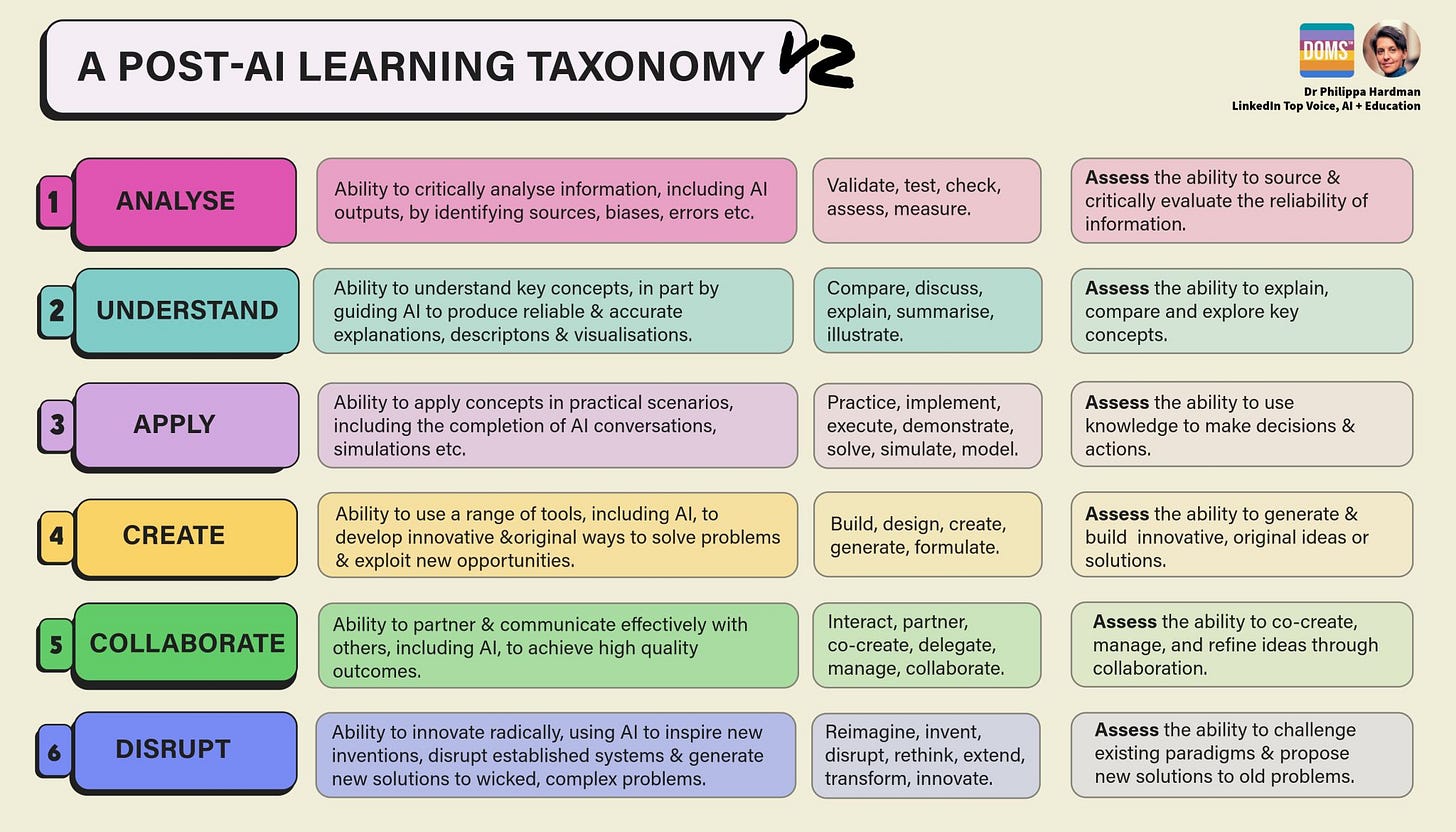
Compared with lectures, essays and quizzes, this new approach to the design, delivery and assessment of learning has far more potential to deliver on what we often say is the primary purpose of education and training: to give us the critical thinking, problem-solving, and collaboration skills we need to be active, fulfilled and productive members of society.
Rather than fearing AI’s role in education, this model embraces it—while ensuring that human judgment, creativity, and ethics remain central to the learning process.
As eduction institutions continue to invest in AI detection technologies, Hodges and Kirschner’s research is a helpful reminder that future of education lies not in finding strategies to circumnavigate AI but in designing new strategies that both transcend and leverage AI’s capabilities to ensure that learners can develop the knowledge and skills that will be essential in a rapidly evolving world.
Happy innovating!
Phil 👋
PS: Get hands-on and explore what post-AI instructional design looks like with me on my AI Learning Design Bootcamp.