A Post-AI Learning Taxonomy
Imagining a New Framework for Designing & Assessing Human Learning
Hey friends! 👋
This week, I've been diving deep into how AI is reshaping our understanding of what learning and the assessment of learning looks like in both higher education and corporate L&D.
One thing that's become crystal clear is that established models of learning - like Bloom's Taxonomy - are struggling to keep up with the impact of AI, both in academia and in the workplace.
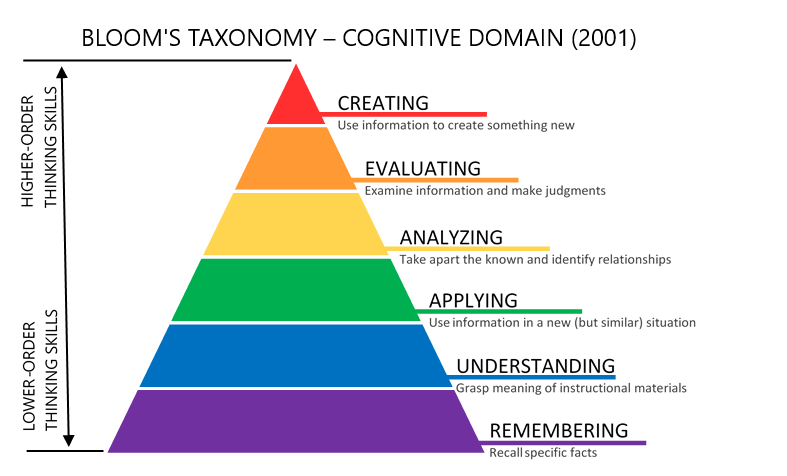
Consider this: in a world where AI can retrieve and present information in seconds, is memorisation still a critical skill? Or should we be focusing on higher-order thinking skills that AI can't replicate - skills that education has aspires to deliver for decades, and which the the World Economic Forum has identified as crucial for the future workforce and global economy?
But here's the exciting part: this challenge is also an opportunity to reimagine how we define and measure “learning” in new and more effective ways.
In this week’s post, I explore some of the problems that AI has presented to establishes systems of learning and assessment and put forward a vision for a new Post-AI Learning Taxonomy that might help us to shift from a state of panic and AI detection to one of experimentation and innovation powered by AI.
Let's go! 🚀
Reframing Fear as Opportunity
Before we dive into the new taxonomy, let's take a moment to consider where we are right now, and why this exercise matters.
The rapid rise of AI technologies, particularly generic AI tools like ChatGPT, has sparked widespread debate in educational and corporate training circles. Many educators and L&D professionals are grappling with big questions like:
Is AI "doing the learning" for students and employees?
Who really wrote this essay / took this quiz?
How do we prevent cheating in an AI-powered world?
These concerns have led some institutions and companies to ban AI tools outright. The core concern often comes down to one question: "Did AI do this work?" And more importantly, if it did, does the learner truly understand and can they really apply the content?
A 2023 Pew survey revealed that a significant portion of K12 educators are still uncertain about AI's role in education (Pew Research Center). This anxiety isn't limited to academia - a recent survey found that 67% of CEOs are concerned about the risks of integrating AI across their businesses, including in learning and development.
Yet this fear overlooks the potential of AI as a powerful tool for guiding learners toward higher-order thinking. Some institutions and organisations are already recognising this, and taking a different approach to the use of AI in teaching and learning.
Many innovative universities, including Arizona State and Harvard University, have already begun rethinking its curriculum to prepare students for a future where AI is a given in the learning process.
At Arizona State University, for example, students have already begun using AI to enhance their learning and assessment processes. In psychology courses, AI tutors are offering students personalised support outside of class, helping them ask better questions and understand complex topics.
Meanwhile, in the corporate world, some large companies are starting to reimagine what their L&D programs would look like if they were to focus on AI literacy and on the development of skills that complement, rather than compete with, AI capabilities.
These initiatives signal the beginnings of an important and, I think, positive shift in focus: as shift from systems of education and training which focus on learners’ ability to memorise and recall information, to a focus on what they can do with information.
TLDR: In a world where AI commodities and automates information retrieval, we need to rethink what learning should look like, whether in a classroom or a boardroom.
A Post-AI Learning Taxonomy
With these challenges and opportunities in mind, I recently asked myself the question: what would a post-AI learning taxonomy look like?
What would it look like to evolve and build on the foundations of established learning frameworks and to create a new approach which emphasises skills that AI enhances rather than replaces - skills that are crucial in both academic and corporate environments.
Here’s where I landed:
Perhaps the first thing you’ll notice is that, unlike Bloom's Taxonomy, this framework doesn't explicitly include a 'Remember' level. In the traditional model, 'Remember' focused on recalling facts, terms, basic concepts, and answers. But in our AI-enhanced world, memorisation can be achieved in more complex and nuanced ways.
In a world where we can access virtually any piece of information in seconds via our smartphones or AI assistants, the value of rote memorisation has significantly diminished.
This doesn't mean that remembering isn't important. Of course, we still need to internalise and retain foundational concepts to be able to learn effectively and deeply. But the emphasis and value of the memorisation exercise has shifted from memorising vast amounts of information to be able to recall it to knowing how to quickly find, verify, and do something with relevant information when needed.
By complicating the process of memorisation to one which requires learners to research, validate and then apply and use what they learn, we make real, substantive memorisation and understanding much more likely.
This doesn't mean that remembering isn't important - it means that the remembering exercise is shifting from memorising vast amounts of information to regurgitate it, to knowing how to quickly find & verify relevant information to do something with it.
In this new paradigm, what's more important is understanding how to guide AI to retrieve accurate information, and then knowing how to use that information effectively. That's why our new taxonomy starts at 'Understand' rather than 'Remember'.
Taxonomy Deep Dive
Let's look at each level of this new taxonomy in detail:
Understand
In this new world, 'understanding' isn't just about absorbing information - it's about guiding AI to produce reliable & accurate explanations, descriptions & visualisations.
Example (Higher Ed): A biology student using AI to create a dynamic, interactive model of photosynthesis, then critically discussing its accuracy with peers using peer-reviewed research data.
Example (Corporate L&D): A sales team using AI to generate market analysis reports, then critically evaluating these reports before using them to understand market trends and customer needs.
Apply
Application now involves using AI as a co-pilot in simulations or real-world scenarios. It's about leveraging AI to enhance our problem-solving capabilities.
Example (Higher Ed): A business student using AI-driven market simulations to apply economic principles in complex, realistic scenarios.
Example (Corporate L&D): A project manager using AI-powered project management tools to simulate different project timelines and resource allocations, applying project management principles to optimise outcomes.
Analyse
Analysis in the AI era is all about critically evaluating information, including AI outputs. It's about identifying biases, errors, and sources in a world awash with AI-generated content.
Example (Higher Ed): A law student analysing AI-generated case summaries to spot potential biases in how different demographics are represented.
Example (Corporate L&D): A HR professional analysing AI-generated candidate assessments, identifying potential biases and ensuring fair hiring practices.
Collaborate
Collaboration isn't just about working with other humans anymore. It's about learning to co-create effectively with both AI and peers.
Example (Higher Ed): A student using AI tools to complete complex projects, e.g. building a start-up using AI Agents.
Example (Corporate L&D): A cross-functional team using AI collaboration tools to brainstorm product ideas, with the AI suggesting connections between ideas and team members refining and expanding on these suggestions.
Create
Creation in the AI era is about using AI as a collaborator to develop truly innovative solutions. It's not about replacing human creativity, but augmenting it.
Example (Higher Ed): An engineering student using AI to help design a prototype for a revolutionary renewable energy solution, pushing the boundaries of what's possible.
Example (Corporate L&D): A marketing team using AI to generate hundreds of ad concepts based on brand guidelines, and evolve these concepts into a groundbreaking campaign.
Disrupt
At the highest level, we're looking at using AI to challenge existing systems and rethink entire paradigms. This is where the real transformative power of AI in education and training lies.
Example (Higher Ed): A healthcare student leveraging AI to propose a completely new model for public health delivery, one that could revolutionise how we approach global health challenges.
Example (Corporate L&D): A team of employees using AI to analyse vast amounts of company data and industry trends, identifying opportunities to completely reimagine their business model for the AI era.
Implications for Education & Corporate L&D
Adopting this taxonomy or one like it could have far-reaching implications for how we think about the design and assessment of human learning:
Curriculum and Training Program Design: We need to shift from content-heavy curricula to ones that emphasise skills like critical thinking, AI literacy, and creative problem-solving - skills that the World Economic Forum has identified as crucial for the future workforce. For instance, IBM has redesigned its corporate training programs to focus on "new collar" skills that complement AI capabilities.
Assessment Methods: Traditional exams and performance reviews that test recall and comprehension no longer hold the same value. We must develop new ways to assess learners' ability to work with AI, evaluate its outputs, and use it to solve complex problems. In the corporate world, this might involve AI-enhanced simulations that assess an employee's ability to use AI tools effectively in realistic work scenarios.
Educator and Trainer Preparation: Educators and L&D professionals need to be equipped not just to use AI tools, but to teach others how to use them effectively and ethically. This might involve professional development programs that focus on AI literacy and pedagogical strategies for AI-enhanced learning environments.
Learning Environments: We need to create learning spaces that facilitate collaboration with both humans and AI, potentially rethinking the physical and digital architecture of our educational institutions and corporate training facilities. This could involve AI-powered smart classrooms or virtual reality environments that allow for immersive, AI-enhanced learning experiences.
Closing Thoughts
Off this back of this exercise, one key takeaway emerged for me: AI has real potential to enable humans to learn more deeply.
How? It does this by encouraging shifting from memorisation and recall to higher-order skills like critical analysis, creative thinking, and problem-solving - skills that higher ed institutions have promised to deliver for decades and which the WEF identifies as crucial for the future workforce.
As educators and L&D professionals, perhaps our biggest challenge is to embrace change - to see the opportunities rather than (or as well as!) the challenges presented by AI.
Instead of asking, “how can we detect who wrote this essay / did this quiz?”, we should start asking: how might we be able to use AI to do a better job of developing the knowledge and skills which are valuable on an individual, organisational and global level?
One thing that gives me hope is that imagining new taxonomies of learning isn't about replacing traditional learning models wholesale - it's about evolving our approach to learning to match the realities of an AI-enhanced world, whether in a university lecture hall or a corporate training room.
What does a post-AI taxonomy look like to you, and how do you see it playing out in your field? I’d love to hear from you!
Happy innovating!
Phil 👋
P.S. If you want to get hands-on experience with AI in a safe and supported environment, join me and other educators and learning professionals on an upcoming cohort of my AI-Powered Learning Science Bootcamp.