The Four Most Powerful AI Use Cases in Instructional Design Right Now
Insights from ~300 instructional designers who have taken my AI & Learning Design bootcamp this year
Hey folks! 👋
As instructional designers increasingly explore if and how to integrate AI into their workflows, a crucial question emerges: what are the most effective AI tools and use cases for instructional design?
Based on extensive testing with ~300 participants and ~50 use cases on my AI & Learning Design Bootcamp, this week I share the four most powerful applications of AI in instructional design today according to designers on the ground.
Let’s go!
1. AI-Powered Analysis: Creating Detailed Learner Personas
One of the biggest challenges in instructional design is getting access to learners for testing assumptions and defining needs. GPT-4 has emerged as a powerful solution, capable (with the right data and prompting) of acting as target learners, enabling us to simulate user interviews to create detailed learner personas.
🚀 Pro Tip: Test AI's ability to embody your learners by first testing it on yourself. Imagine you’re the target learner and have AI interview an AI version of you - how well does it assess and capture your needs? This helps validate how accurately it predicts responses and behaviours, and provides insights into how to edit your prompts to get more reliable outputs.
2. AI-Powered Design: Optimising Instructional Strategies
When it comes to selecting the optimal instructional strategy for specific learners and goals, AI-powered research tools are revolutionising our industry. These tools provide unprecedented access to robust information about how to effectively teach specific concepts and skills to specific types of learners in specific contexts.
According to designers on the ground, the standout tool in this category is Consensus - think of it as ChatGPT but specifically trained on trustworthy, peer-reviewed research. It's become an invaluable resource for evidence-based instructional design decisions.
🚀 Pro Tip: Try the Consensus GPT in ChatGPT - it's like having a personal research assistant at your fingertips.
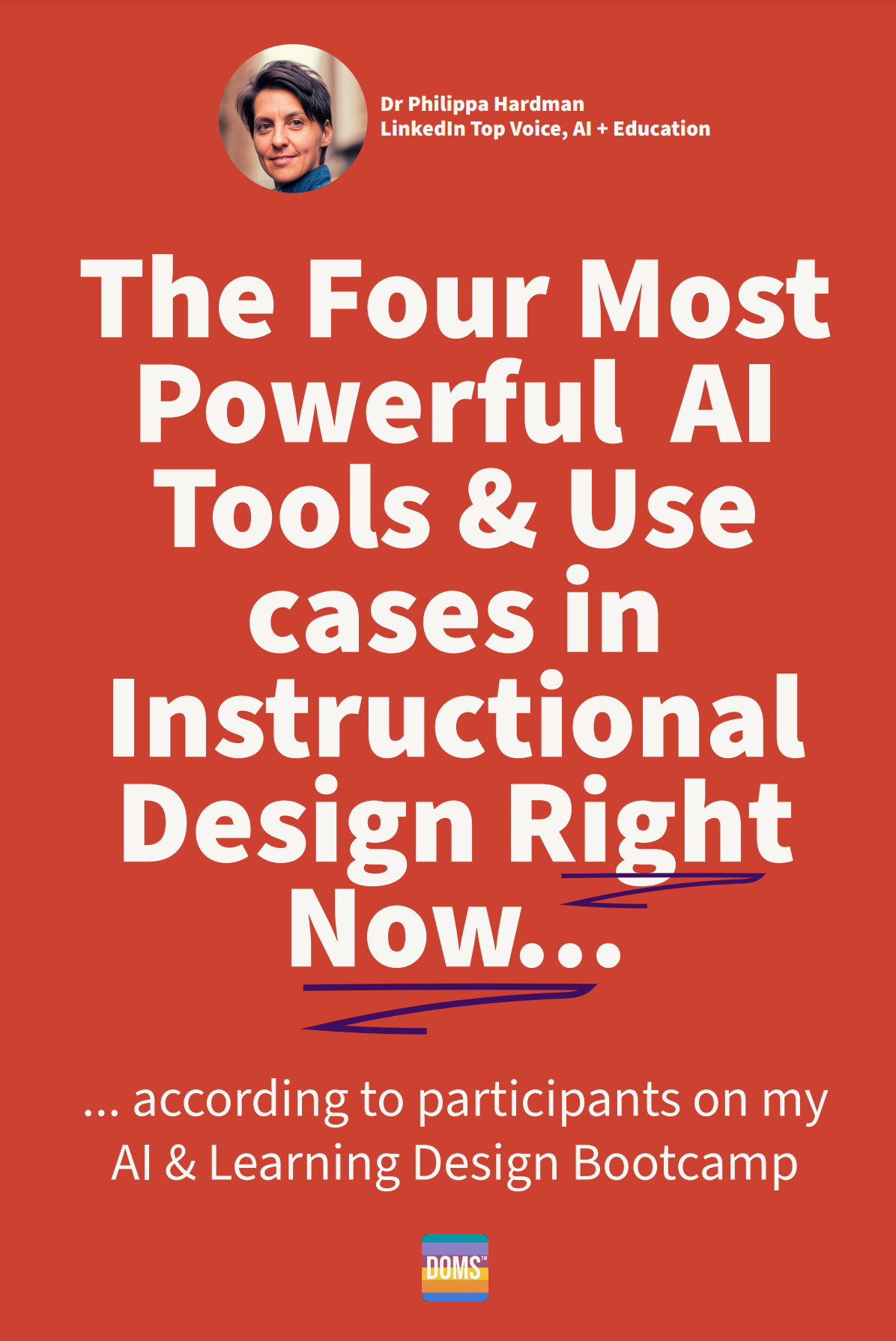
3. AI-Powered Development & Implementation: Quality Assurance
AI excels at pattern recognition, making it particularly valuable for quality assurance in instructional design. One key application is checking for alignment with WCAG, UDL, and other quality requirements and standards.
Claude has proven particularly effective in this role: with proper prompting, it excels at identifying both alignment and misalignment with defined standards.
🚀 Pro Tip: Don't get fixated on AI-powered content creation during the development phase. AI's capabilities extend far beyond generating videos and images.
4. AI-Powered Evaluation: Predictive Impact Assessment
Testing and iterating learning designs is crucial for impact, but it's often impractical due to time and resource constraints. GPT-4 offers a solution by generating feedback on your course's likely impact before you build or deliver it.
The tool's ability to predict human responses and behaviours using basic learner data makes it invaluable for preliminary evaluation and iteration.
🚀 Pro Tip: Expand your AI role-playing scenarios to include stakeholder reactions, not just learner responses.
Conclusion
These tools represent the cutting edge of AI application in instructional design, but they're just the beginning. As AI technology continues to evolve, its potential to enhance learning design grows exponentially. The key is understanding not just how to use these tools, but when and why to deploy them for maximum impact.
One thing I encourage and help designers practice on my bootcamp is a research mindset. To do this:
Define a Theory of Change: Write a hypothesis about the impact that you expect AI to have on a) the speed and b) the quality of the instructional design task you’re testing it with. Example:
Hypothesis: Using Claude to assist with the development of clear, measurable learning objectives will:Speed: Reduce the time required to create a set of learning objectives by 60%.
Quality: Improve the specificity and alignment of learning objectives with desired outcomes, increasing peer-review ratings from an average of 3.5/5 to 4.5/5.
Measure Impact: Find simple ways to measure the impact of using AI on a) the speed and b) the quality of the task you’re testing it with compared with your existing process. If you’re not sure how to do this, ask Claude or ChatGPT!
I’d love to hear your thoughts on this and any experiments you run. Join the conversation via the related LinkedIn post here.
Happy experimenting!
Phil 👋
PS: Want to get hands-on and learn how to integrate AI tools into your instructional design work? Apply for a place on my AI & Learning Design Bootcamp to master new techniques and hone your skills.
PPS: Want to share and keep these use cases? You can download a copy of the PDF from my Gumroad site.