The AI Sounding Board
Can AI act as proxy for learners & business leaders in the instructional design process?
Hey folks! 👋
A number of recent studies, including a very recent piece of by Hewitt et al. (2024) at New York University and Stanford, have shown that GPT-4 can predict human responses with a correlation coefficient of r = 0.85 (spoiler: that’s huge!).
What it means in practice is that AI is getting very good at simulating human behaviour and reactions.
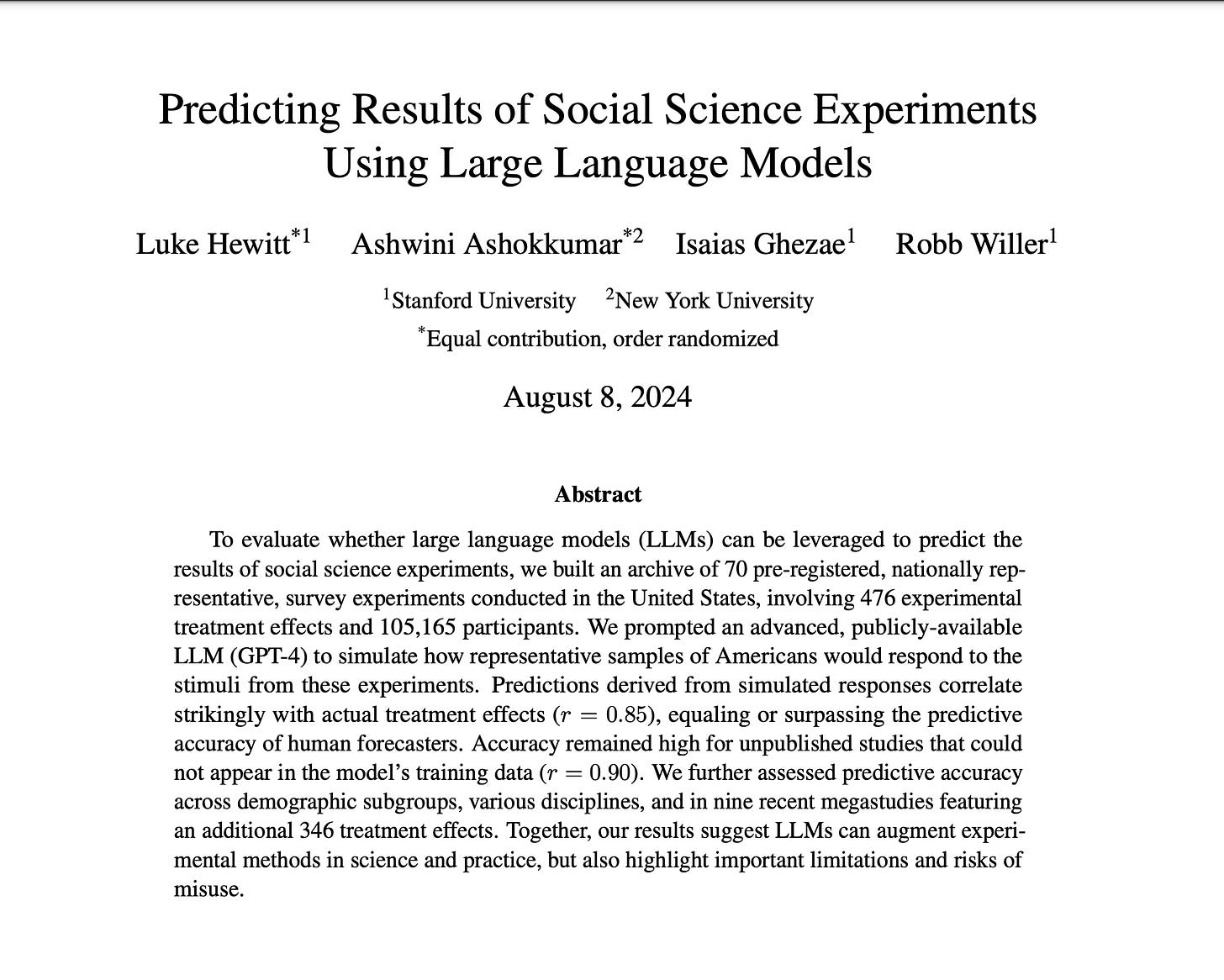
This got me thinking: can AI “act” as key stakeholders in the instructional design process? Imagine a world where, with the right inputs, AI can reliably take on the role of business leaders and target learners for infinite conversation about the problems we're trying to solve and the best-fit solutions.
The answer is: yes, with some important caveats.
In this week’s blog post, I’ll spin through what we’ve learned so far from research in this space, apply it to each stage of the instructional design process and share some practical experiments for you to try.
Let’s go!
Analysis Phase: AI as Your Focus Group & Executive Team
As instructional designers, we all know that feeling when you're trying to understand learner needs and business objectives, but you can only talk to a small handful of people for a quick 30 mins grabbed here and there.
What if you could use AI to simulate responses from hundreds of learners and dozens of executives in minutes?
Hewitt et al. (2024) found that GPT-4 can reliably predict human responses. In practice, this means that AI could potentially simulate a large number of stakeholder perspectives with high accuracy.
Imagine a world where we “interview” a potentially infinite number of learners and business leaders to go deep on problem and solution definition.
Here’s what this could look like in practice:
Create 5-10 learner personas and 3-5 business leader personas
For each persona, ask ChatGPT: "You are a [insert persona details]. What are your top 3 learning needs/business objectives regarding [specific skill/topic]?"
Ask follow-up questions to dig deeper
Compare AI-generated responses with actual data from past needs assessments or a small sample of real stakeholders
Analyse the similarities and differences
Risks & Mitigations
Lack of response variance: Bisbee et al. (2024) found that AI-generated responses often showed less variation than human responses. This could lead to missing nuanced or emerging needs. Mitigation: Compare AI responses across multiple runs and deliberately probe for outlier perspectives. Supplement with real stakeholder input where possible.
Psychometric limitations: Petrov et al. (2024) identified poor psychometric properties in AI-generated responses, especially for complex psychological traits. Mitigation: Focus on concrete needs and objectives rather than personality-based insights. Validate any personality-related findings with established psychometric tools and real stakeholder data.
Demographic biases: While Hewitt et al. (2024) found high predictive accuracy across different demographics, there's still a risk of perpetuating or amplifying biases present in the AI's training data. Mitigation: Explicitly include diverse demographic profiles in your prompts and cross-reference AI responses with real data from underrepresented groups.
Design Phase: AI as Your Target Learner & Strategy Team
Imagine being able to test multiple instructional design approaches with simulated learners and get instant feedback from “virtual executives”.
Studies by Hewitt et al. (2024) and Bisbee et al. (2024) suggest that AI is capable of effectively simulating learner engagement with different instructional designs, providing us with an opportunity to rapidly test and get feedback on proposed solutions.
Here’s what this might look like in practice:
Draft a course outline including learning objectives
Prompt ChatGPT: "As a [learner/business leader], review this course outline and objectives. What seems most relevant/irrelevant to you? What's missing? How does this align with business goals?"
Ask follow-up questions to dig deeper
Compare ChatGPT's analysis with your own assessment and, where possible, real stakeholder feedback
Risks & Mitigations
Oversimplification: Bisbee et al. (2024) noted that AI tends to generate more standardised responses, potentially missing nuanced learner needs or complex business contexts. Mitigation: Use AI feedback as a starting point, but actively seek out edge cases and complex scenarios in your prompts. Validate key design decisions with real stakeholders.
Text-based bias: Hewitt et al. (2024) found AI predictions were more accurate for text-based interventions, potentially leading to an overemphasis on text-based design elements. Mitigation: Explicitly prompt the AI about non-text elements (e.g., "How would visual or interactive components enhance this objective?"). Always prototype and test non-text elements with real users.
Interaction effects: Hewitt et al. (2024) noted limitations in predicting heterogeneous effects when subgroup differences were present. Mitigation: Prompt the AI to consider how different learner groups might interact with the content differently. Use these insights to design more inclusive learning experiences, but validate with real diverse user groups.
Development & Implementation Phase: AI as Your Beta Testers & Executive Reviewers
Hewitt et al. (2024) demonstrated AI's capacity to simulate learner interactions with content, predicting how different demographics might react to different materials.
For instructional designers, this could be a game-changing way to get instant feedback on our content from AI versions of our learners and business leaders, allowing for quick adjustments in tone, complexity, or case studies.
Here’s what it might look like in practice:
Develop a sample of your course content
For each project persona, ask ChatGPT: "You are a [learner/business leader]. Review this content and identify [insert what you want to test, e.g. main points that you understood, irrelevant confusing parts, alignment with business objectives."
Use insights to improve content and activities. Where possible, compare AI responses with sample feedback from actual stakeholders
Risks & Mitigations
Limited feedback on non-text content: Hewitt et al. (2024) found lower accuracy for non-text-based interventions, suggesting AI may struggle with multimedia or interactive elements. Mitigation: Provide detailed descriptions of non-text elements in your prompts. Use AI feedback as a starting point, but prioritise real user testing for multimedia and interactive components.
Lack of context: Petrov et al. (2024) raised concerns about AI's ability to account for the full learning context and learner background. Mitigation: Provide rich contextual information in your prompts, including learner backgrounds and organisational context. Cross-reference AI insights with subject matter experts and real learner feedback.
Overconfidence in predictions: Bisbee et al. (2024) noted that raw AI predictions often overestimated actual effects. Mitigation: Treat AI’s outputs as hypotheses to be tested, not definitive answers. Always validate key insights with real stakeholders and pilot testing.
Evaluation Phase: AI as Your Survey Respondents and ROI Analysts
Imagine being able to run a course evaluation and Return on Investment analysis before your course even starts. This a dream that many instructional designers have discussed for decades and one that could become a reality in a post-AI world.
Bisbee et al. (2024) found that AI can effectively predict behavioural and material outcomes based on profiles and goals. Despite limitations (see risks and mitigations), the research so far indicates that AI can already reliably provide rapid, initial insights to guide more targeted human-led evaluations.
Here’s what this might look like in practice:
Design a post-course evaluation survey and ROI assessment
Create 10-15 learner personas and 3-5 business leader personas
For each persona, ask ChatGPT to complete the survey/assessment: "You are a [learner/business leader] who just completed/implemented this course: [Describe course]. Complete this evaluation/ROI assessment from your perspective."
Analyse AI-generated responses as you would real data
Ask follow-up questions to probe how AI has come to its conclusions (if in doubt, ask why - and then ask why again)
Compare insights with actual course evaluation and business impact data, if available
Risks & Mitigations
Overestimation of effects: Bisbee et al. (2024) observed that AI tends to overestimate effect sizes, potentially leading to overly optimistic evaluation results. Mitigation: Apply a correction factor to AI-generated effect size estimates based on comparisons with historical data. Use AI insights to identify trends and patterns, but rely on real data for precise measurements.
Limited capture of unexpected outcomes: The lower variance in AI responses (Bisbee et al., 2024) might mean missing unexpected but valuable feedback. Mitigation: Explicitly prompt the AI for "unexpected" or "surprising" outcomes. Design your real evaluation process to capture open-ended feedback and qualitative insights that AI might miss.
Psychometric concerns: Petrov et al.'s (2024) findings suggest AI-generated evaluation responses might not accurately reflect underlying constructs being measured. Mitigation: Focus AI-generated insights on concrete, observable outcomes rather than complex psychological constructs. Validate any psychometric-based findings with established tools and real participant data.
Conclusion: AI as Sidekick
So, what's the bottom line? 🤔
AI, especially advanced models like GPT-4, offers exciting possibilities for simulating stakeholder input and feedback throughout the instructional design process. By acting as proxy learners and business leaders, AI can help us gain deeper insights into both the problems we're trying to solve and the potential effectiveness of our solutions.
However, here’s the key takeaway: all research so far has concluded that AI should augment our user research and testing processes, not replace them. According to all studies so far, the most effective approach is that which combines AI-generated stakeholder insights, human judgment, and real stakeholder input.
All research so far has concluded that AI should augment our user research and testing processes, not replace them.
So, can AI truly "act" as stakeholders to give us a deeper understanding of our design challenges and solutions?
Based on the current research, the answer is a cautious "yes, but..." AI shows remarkable potential in simulating stakeholder responses, but it comes with limitations and risks that we human instructional designers need to carefully navigate.
To make the most of AI as a proxy for human stakeholders in your instructional design process:
Use AI as a brainstorming and hypothesis-generation tool
Always validate key insights with real stakeholders
Be aware of AI's limitations and biases
Combine AI-generated insights with your expertise and real-world data
Continuously evaluate and refine your AI prompts and processes
I'd love to hear your thoughts on this. Have you tried using AI to simulate stakeholder input in your instructional design process? What challenges have you faced? Drop your comments in the related LinkedIn post, and let's start a conversation!
Happy experimenting! Phil 👋
P.S. If you want to get hands-on and integrate AI into your instructional design process check out my AI Learning Design Bootcamp.