The AI Magpie Effect
The existential risk of text-to-content tools for learning designers, educators & learners
This week, we saw another round of news releases about AI products that will soon come to market. Among them was Open AI’s news about Sora, a text-to-video tool, and Articulate’s news release about a set of new AI features.
Both of these products are the latest in a string of text-to-video AI tools which make it faster and easier for instructional designers to make content, primarily, video, more quickly.
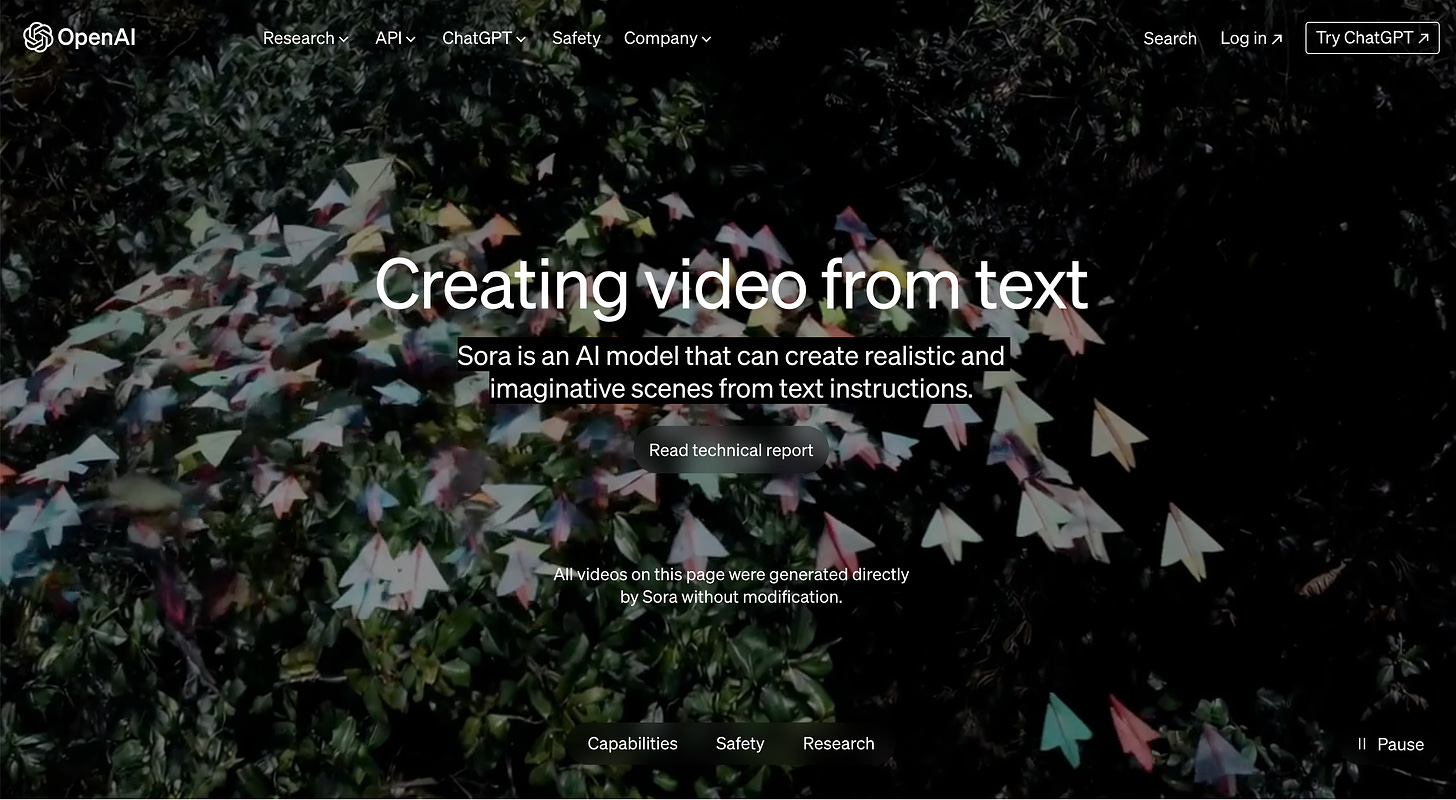
Sora is, as OpenAI, describe it, “An AI model that can create realistic and imaginative scenes from text instructions”.
While Articulate does more than just create video, its promo video shows that the feather in Articulate’s cap and the intended “aha” moment of the product is its ability to turn text into video at the touch of a button.
For learning designers who spend days, maybe weeks, maybe even months working with production teams and film crews to create video, AI-powered text-to video tool which “make creating video as quick and easy as making a slide deck” do indeed feel like a bit like magic (or, failing that, a very effective painkiller).
Sora and Articulate AI come hot on the heels of popular tools like Synthesia, Colossyan and other text-to-video products which, thanks to their ability to make video creation considerably cheaper and faster, have become increasingly popular with learning designers over the last last few months.
Increasing the speed at which we can create video content is good news for learning designers and the learners they serve, right? Well, maybe.
As more and resource is invested in AI-powered content creation tools, a memorable quote from Prof. Dan Schwartz looms large in my mind: “the biggest risk of AI is that it makes us more effective as ineffective practices.”
My perhaps unpopular opinion is that, so far, we have significantly under-utilised AI in the world of education.
Generative AI tools which turn text into video, images, audio, slides etc are designed to solve a practical problem: the speed and cost of content creation . Meanwhile, a more fundamental and wicked problem looms large: video + quiz courses that we can now create in record time are unfit for purpose.
The average completion rate of a traditional video + quiz style online course flat lines somewhere at ~3%. Last year, $300 billion was spent on video + quiz style online learning in corporate L&D alone, but only 12% of employees reported any sort of impact on their knowledge and skills. Meanwhile, a lack of effective training remains the #1 reason that employees leave their jobs.
The average completion rate of a typical, long-form video + quiz style online course flat lines somewhere at ~3%.
My call to action is that before we think about how to use AI to accelerate the speed at which we build learning experiences, we need to ask two more difficult but also important questions:
How can we use AI to understand what sort of learning experiences should we be building?
How can we use AI to help us to design, deliver and evaluate learning experiences which are capable of achieving our ultimate goal - to positively and measurably impact the knowledge, skills and behaviour and of learners?
The answer to these question is complex, but here are some answers which are emerging from my research:
AI-Powered Problem Identification
At its most basic, AI is a data machine: it can “consume”, analyse and synthesise large huge quantities of data and help us to make more powerful data-informed decisions than we could ever make alone.
In the corporate learning context, what if we used AI to analyse performance data, identify performance gaps and define L&D training priorities based on the highest-value interventions, mapped to the most important KPIs?
What if we were to use Natural Language Processing (NLP) to run sentiment analyses on employees’ qualitative feedback and informal conversations to identify areas of struggle and success and used this to inform what we sort of training we design and how we design it?
AI-Powered Solution Identification
Once we’ve identified the problem, what if we were to use AI to run root cause analysis: is the problem due to a lack of knowledge and skills?
Or is it a motivation issue, a process or tool issue or a communication issue? Put simply, by analysing data we can ask an all-important question is rarely asked: is this actually a course or training? Or does the solution lie in a different sort of solution?
AI-Powered Design
Once we’ve identified that the solution is a training, what if we were to work wit AI to find the best possible way to deliver the knowledge and skills that our learners need in order to hit our goal?
What if instead of defaulting to video + quiz or expensive in-person gatherings, we could search are databases of learning science research and existing course data to make data-informed decisions about things like instructional strategies, modes of delivery and content formats?
As more and more investment is made by both venture capitalists who build AI ed-tech products and organisations who buy them, it’s important that we don’t get distracted by the magic of tools which only serve immediate pain.
By keeping our eye on the most immediate prizes and being seduced by the magpie effect of text to content tools, we ignore and deprioritise Ai use cases and innovations which have much more potential to transform the quality and impact of education.
I’d love to hear your thoughts on this! If you have questions, you can tag me on LinkedIn.
Happy designing,
Phil 👋
PS: If you want to learn more about how AI is impacting how we design, deliver and evaluate learning experiences, check out my AI Learning Design Bootcamp and my monthly Learning Futures newsletter.