The AI-Education Divide
How the rise of AI has reinforced inequity in education (and what we need to do to reverse it)
AI has often been hailed as a powerful tool for the democratisation of education.
Many of us who work in the world of AI & education are motivated by the vision that AI can make better education available to more students and, in the process, increase social mobility and equity.
Millions of students around the world are already starting to benefit from the use of AI in education, but millions more are not.
My initial research suggests that just six months after Open AI gave the world access to AI, we are already seeing the emergence of a significant AI-Education divide.
If the current trend that continues, there is a very real risk that - rather than democratising education - the rise of AI will widen the digital divide and deepen socio-economic inequality.
In this week’s blog post I’ll share some examples of how AI has impacted negatively on education equity and - on a more positive note - suggest some ways to reverse this trend and decrease, rather than increase, the digital and socio-economic divide.
🚀 Let’s go!
Part 1: Algorithmic Bias & the AI Divide
At its most basic, AI is a machine that finds patterns in data and uses those patterns to make predictions, decisions or (in the case of generative AI) to generate new information. This is what we refer to as Machine Learning.
In this process, humans first select and feed the program data. Then, we ask the programme to identify patterns and relationships in the data - this is what were refer to as algorithms.
For example, let's say you want to build an AI programme dedicated to cats (because, who wouldn’t?).
You would first train the program on a dataset of information about cats, e.g. a set of images. The program would analyse the images and learn the unique distinguishing features of a cat, like the shape of their ears, their fur colour, or their tails.
Once the program has learned these features & patterns, it can then “recognise” cats in new data and, in the case of Generative AI, generate new images of cats based on the patterns it’s learned.
Of course, AI doesn’t know what a cat is: it just predicts that something is a cat based on what it recognises as distinguishing features of cat, which is based in turn on the data that we humans feed it.
Which brings me to the first big risk with AI: algorithmic bias.
AI is only as reliable as the data it’s trained on, and that data is both incomplete and biased.
AI is only as reliable as the data it’s trained on, and that data is both incomplete and biased.
AI learns from patterns in data that we feed it. If a certain piece of data wasn’t part of that training data, the AI will not be able to handle it accurately or effectively.
For example, if our AI has been trained only on data about cats, and you ask it about dogs, it won't be able to give you a useful answer because it doesn't have any input data about dogs.
What this means is that AI reproduces human blindspots in two ways:
It reflects back only what we consider to be “important data” (incompleteness)
It reflects back cultural, political and societal perspectives which exist in the data that we have created over the last X years (bias)
For example, if an AI is trained on data from job applications and the data shows (because of cultural, political and societal biases) a bias towards hiring middle class men for leadership roles, the AI will learn to associate men with leadership roles, even if a woman or someone from a different social background is equally or more qualified.
In the world of education, this can have incredibly harmful results. Take, for example the UK A-Level results scandal of 2020.
In the wake of COVID-19, it was decided that instead of humans grading exams, an AI algorithm (so, a prediction based on inputted data) would be used to predict students grades. In theory, this would make the process faster and eliminate human subjectivity. In practice, the outcome was very different.
The AI was asked to base student grades not just on individual student performance, but also longer term whole-school performance. This meant that if you were a really hard-working student but your school hadn't performed well in previous years, the AI algorithm predicted a lower grade for you.
Long story short, the AI reproduced human biases and blindspots.
Bigger picture, there are a number of ways in which algorithmic biases could have a significant negative impact on the world of education:
Reinforcing existing educational disparities: Algorithmic bias can perpetuate and reinforce existing educational disparities. If AI systems are trained on biased data that reflects historical inequities in education, they may inadvertently perpetuate those biases. For example, if an AI-based system for evaluating student performance is trained on data that reflects biased grading practices or discriminatory disciplinary actions, it may unfairly disadvantage certain groups of students, such as students from marginalized communities or students with disabilities.
Limiting access to educational opportunities: AI systems can contribute to limited access to educational opportunities. If algorithms are biased in their decision-making processes, they may inadvertently discriminate against certain individuals or groups. For instance, if an AI-powered system is used for college admissions and it is trained on biased historical admissions data, it may disadvantage students from underrepresented backgrounds or those attending schools with fewer resources. This can perpetuate inequities and hinder social mobility.
Narrowing the curriculum: Algorithmic bias can also result in a narrowing of the curriculum. If AI systems are trained on data that reflects limited perspectives or biases in educational content, they may reinforce existing biases and limit the diversity of perspectives and knowledge presented to students. This can result in a skewed representation of history, culture, and societal issues, leading to an incomplete and biased understanding of the world.
Unfair allocation of resources: AI-powered systems are increasingly being used for resource allocation in education, such as determining funding distribution or identifying students in need of additional support. If these systems are trained on biased data, they may perpetuate existing resource disparities. For example, if an algorithm is trained on historical funding data that reflects biased distribution practices, it may allocate resources in a way that disadvantages schools or districts serving marginalised communities.
Perpetuating stereotypes and bias in career guidance: AI systems are often used for career guidance and recommending educational pathways. If these systems are trained on biased data, they may perpetuate stereotypes and bias in career recommendations. For instance, if an AI system is trained on data that reflects gender or racial biases in career choices, it may steer students towards or away from certain fields based on those biases, limiting their opportunities and reinforcing societal stereotypes.
So what can we do about this? Your call to action:
Develop critical awareness: Educators should develop a critical awareness of the potential biases in AI systems and the impact they can have on students. Stay informed about the limitations and risks of AI technologies in education, including algorithmic bias. This awareness will enable educators to make more informed decisions when using AI-powered tools and resources.
Evaluate AI tools and resources: Before incorporating AI-powered tools or resources into their teaching practice, educators should thoroughly evaluate them for potential biases. Consider factors such as the diversity and representativeness of the training data, the transparency of the algorithms used, and any available evidence of fairness and accuracy. Look for tools and resources that prioritize equity and inclusivity.
Advocate for diverse and inclusive AI: Educators can advocate for the development and use of AI systems that are designed to be diverse, inclusive, and free from biases. Engage in conversations with administrators, policymakers, and technology providers to highlight the importance of fairness, equity, and transparency in AI tools and systems. Encourage the adoption of ethical guidelines and policies that promote unbiased AI in education.
Use multiple sources of data and perspectives: Avoid overreliance on AI systems and algorithms as the sole source of information and decision-making. Incorporate multiple sources of data and perspectives in educational practices. Encourage students to critically analyze and question the information provided by AI systems, promoting a well-rounded understanding of the subject matter.
Provide counter-narratives and diverse perspectives: Actively seek out and include diverse perspectives, experiences, and voices in your teaching materials and discussions. Use inclusive pedagogical approaches that challenge stereotypes and biases. Encourage students to question and analyze the information presented by AI systems and to consider alternative viewpoints.
Promote digital literacy and critical thinking: Teach students about the potential biases and limitations of AI systems. Help them develop digital literacy skills to critically evaluate the information and recommendations provided by AI tools. Foster a culture of critical thinking, where students question and examine the outputs of AI systems rather than accepting them uncritically.
Collaborate with other educators: Engage in professional collaboration and share insights and best practices with other educators. By working together, educators can collectively address algorithmic bias and promote fair and equitable practices in the use of AI in education.
Part 2: AI Expertise & the AI Divide
A second potentially concerning trend that has emerged over the last couple of months or so is growing inequity of access to AI tools & expertise.
In response to what the World Bank described as, “governments’ longstanding failure to create effective frameworks for learning technologies in education systems”, very few non-fee paying schools globally have comprehensive strategies for how to integrate technology in general and AI in particular into education systems.
This means, of course, that state-funded education systems are vulnerable to the rapid leaps now being seen in the capability and widespread availability of AI tools elsewhere in the system.
Cut to the independent education sector.
Earlier this month, Cottesmore School - a notable independent boarding school in the UK - broke new ground by advertising for a Head of AI. Their role? To lead the integration of AI technologies and educational strategies into the school’s teacher training programme and student curriculum.
This followed an international AI conference hosted at the school and headlined by AI experts from around the world to discuss and make recommendations on AI and learning technologies in schools. Another will follow in September.
With resource and independence, a growing number of independent schools like Cottesmore and related organisations like the Independent Schools Council and Global Independent Schools Association (GISA) are working closely with world-leading experts to lead the way in AI powered teaching and learning.
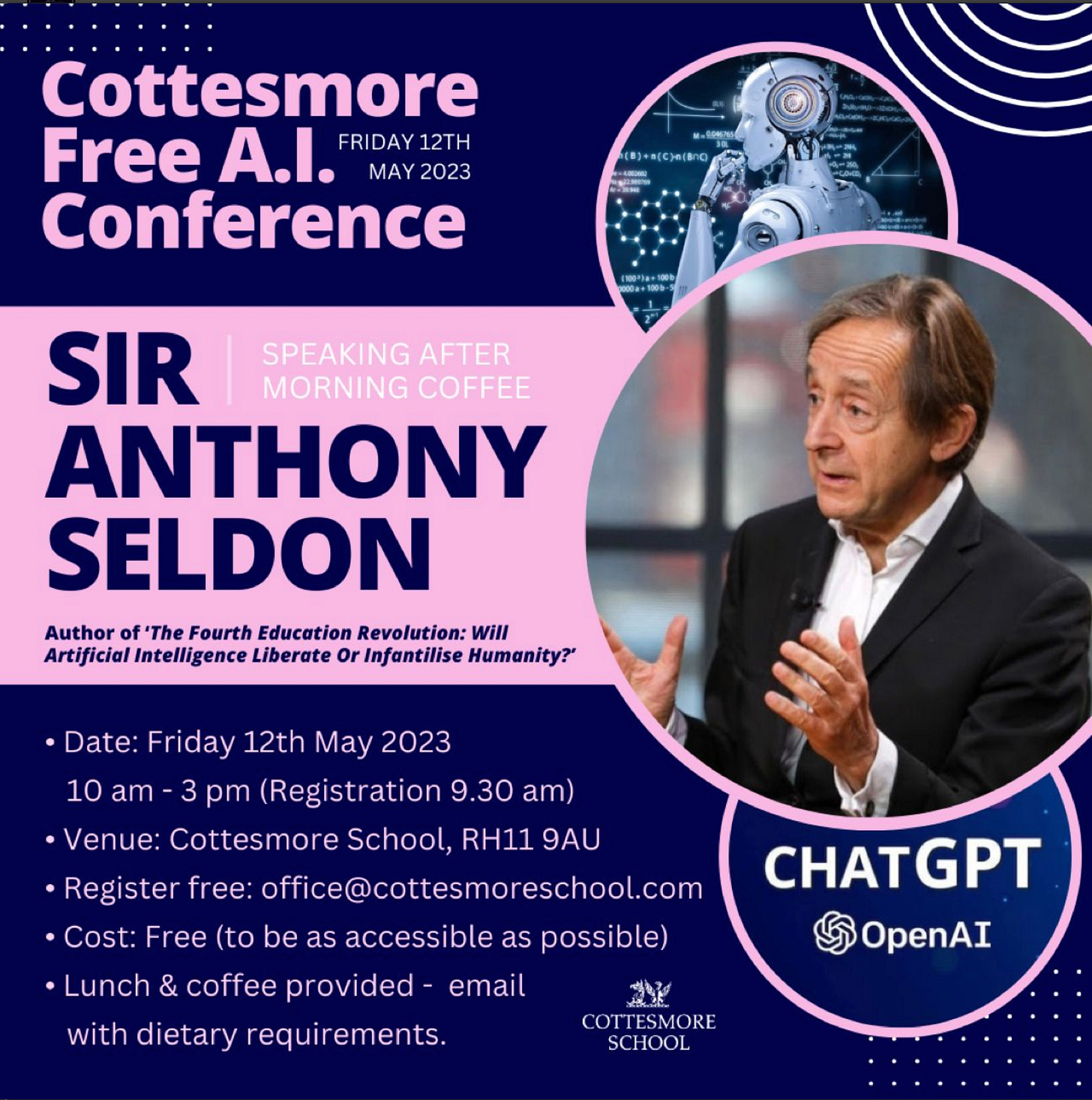
This has implications for both equity of education and, related to this, equity of access in the workplace.
As the WEF’s Future of Jobs Report 2023 recently reported, the most likely future is one where AI literacy and machine learning skills are at a premium, making AI education a new and valuable currency and making pupils who don’t develop these skills vulnerable not just to inequity in education but also - and as a direct result - in access to employment.
The majority of the fastest growing roles are technology-related roles. AI & Machine Learning specialists top the list of fastest-growing jobs.
WEF Future of Jobs Report, 2023
What this means is that wealthier students are likely to gain additional advantages over less wealthy students not within the educational system but also, as a result, the workforce.
The same concern has been raised repeatedly in recent weeks by a number of prominent educators in Australia, where the use of AI has been banned in state-funded schools but allowed in private schools, raising fears that private school kids are gaining an unfair edge within a new, AI-education divide.
The key takeaway here is that if we fail to act quickly and decisively, AI has the potential to broaden the digital divide and exacerbate social and economic inequality in the UK and globally.
So, what can we do?
Build AI-education programmes: if we want to mitigate the risks of the AI-education divide, we need to ensure that all teachers have access to high quality training. This requires concerted effort by both governments and school leadership teams.
Start to learn about AI: while it’s not comprehensive, some high quality, free AI information and training is already available. Many of the conversations happening in the independent sector, for example, are free for all educators to attend. DeepLearning also offer high quality AI and Machine Learning courses for non-specialists.
Be part of the conversation: governments, including the Department for Education in the UK, are starting to think about what AI might mean for educators and the education system. Be part of the conversation and help emphasise the need for urgent and systematic support for educators in a post-AI world.
Conclusion
AI could, without a doubt, help to make education systems more effective, equal and fair.
We (and especially governments) often talk about AI as a golden bullet which - by giving learners access to personalised learning pathways and AI coaches - will solve the attainment gap and increase social mobility and equity.
The reality is very different.
The independent education sector is already significantly further ahead than the state sector, putting wealthier students at an unfair advantage in the workplace.
It’s also notable that the majority of learners who are using and benefitting from “outside school” AI tools like Khanmigo’s AI teaching support are middle class kids who have access to an internet connection and also to the confidence and literacy required to request help and respond to it.
AI is not automatically a force for education equity.
If we really want to leverage AI to democratise education and give everyone the same life chances, we need to build it and implement it very intentionally with this goal in mind.