Learning Design in the Era of Agentic AI
Aka, how to design online async learning experiences that learners can't afford to delegate to AI agents
Hey folks,
The rapid emergence of agentic AI has forced the learning and development field to confront a long-standing truth: most asynchronous online learning is not well designed.
Last week, I analysed how AI-driven agents like Manus are autonomously completing traditional online courses—moving through static content, passing quizzes and even meaningfully contributing to online discussions.
The point I put forward was that the problem is not AI's ability to complete online async courses, but that online async courses courses deliver so little value to our learners that they delegate their completion to AI.
The harsh reality is that this is not an AI problem — it is a learning design problem.
However, this realisation presents us with an opportunity which we overall seem keen to embrace. Rather than seeking out ways to block AI agents, we seem largely to agree that we should use this as a moment to reimagine online async learning itself.
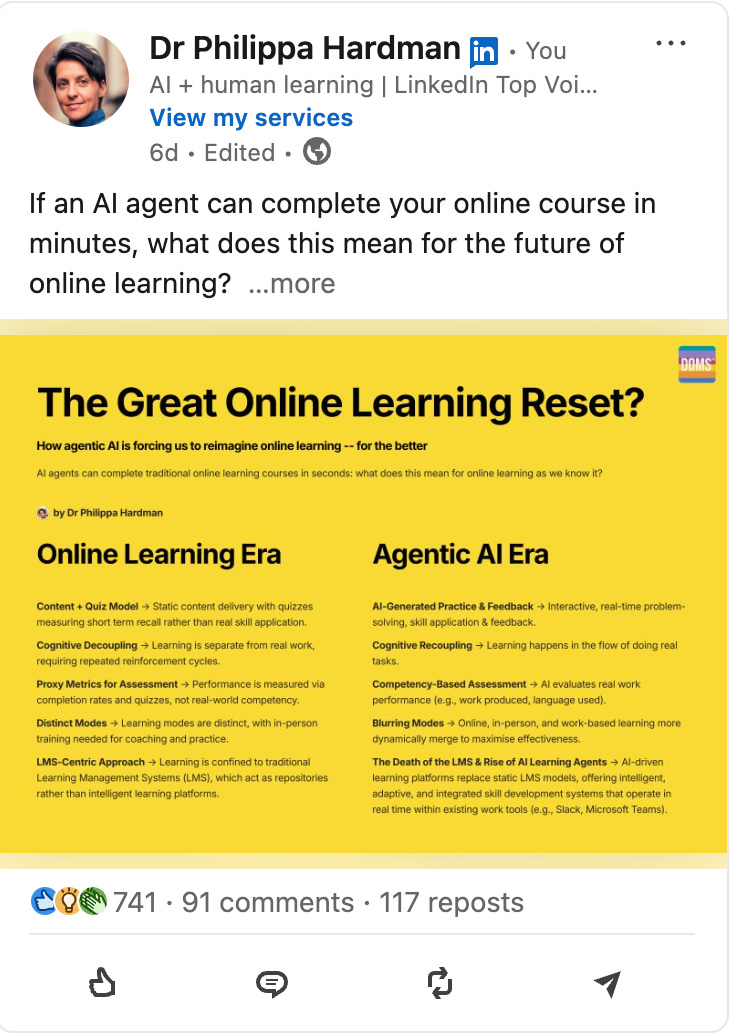
So what might this look like in practice? In the medium to longer term I anticipate a pretty wholesale reset and re-imagining of how we design, develop, deliver and evaluate online learning.
But what about the immediate term? In a world where we continue to be limited by the capabilities of elearning authoring tools and LMSs, how do we start on a journey towards more relevant, valuable and impactful online learning experiences?
I think the answer is three-fold:
Analysis: Rethinking how we analyse learning needs, shifting focus from org and educator-first analysis, to learner-first analysis)
Design & Development: Redesigning content and activities to prioritise active decision-making over passive consumption and recall.
Evaluation & Iteration: Transforming evaluation methods to measure real-world application rather than mere completion rates.
Let’s dive into this in more detail.
The Three Shifts Required for Effective Async Online Learning
1. Analysis
The Problem: Organisation & Educator-Centred Learning Goals
Most instructional designers start by defining what the company and/or educator needs and wants their learners to know.
Overwhelmingly, when we ask “what sort of online learning we designing, and why?” the answer is centred on the needs and wants of the business or educator, not the learner.
This results in learning experiences which feel abstract, generic and compulsory. Without a clear and compelling value proposition for the learner about what’s in this for them, and without an offer that is deeply relevant, motivation will only ever be extrinsic (i.e. learners complete online courses because of a stick, not a carrot) and as a result any “learning” that takes place will at best amount to short term recall.
Time once again for my favourite ever TikTok:
The Shift: Learner-First Course Design
A more effective approach is to centre the learner and ask: what’s in this for them? What will motivate them to engage with this learning experience, and what will demotivate them?
I’ve written before about how designing learning experiences is a lot like designing products. When we start the learning design process, we need to recognise that we may well want to build X for Y goal, but if we build it will our learners come? Do they care? What’s in it for them? What makes this worthy of their time and energy?
By asking these learner-centred questions and exploring our learners’ psychographics, we generate the data we need to be able to design learning experiences in a way that drives intrinsic motivation to learn, which in turn supports engagement and substantive learning.
Example: Traditional vs. Optimised Course Framing
Imagine you’re an employee at GSK, required to take an online course on their new Misconduct Reporting processes.
2. Design & Development
The Problem: Passive Consumption of Information
Most online courses rely on content-first, test-later approaches and structures: learners watch a video or read static text, then complete a multiple-choice quiz. The result?
Minimal engagement: learners are passive recipients of information, they do not practise applying their knowledge (Choi & Hur, 2023)
Low retention: reasons to “stay and learn” are low, leading to low retention and completion and the need to make training compulsory (Bawa, 2016; Muilenburg & Berge, 2001)
Zero transfer: where there is only content and knowledge checks, the best we can hope for is the ability to recall concepts in the right way for a short time with minimal real world impact for learner or org (Zhao et al., 2024; Liyanage et al., 2022)
The Shift: Active, Scenario-Based Learning
Instead of focusing on knowledge-transfer models, when designing online async courses try to follow the Problem-Solution-Instruction (PSI) model. In this model:
Problem: Learners are thrown straight into the action by encountering a real-world problem, dilemma or similar.
Solution: Using supporting content, learners take an action or make a decision and receive guided feedback.
Instruction: In the context of their experience, learners get instruction to explain what great looks like and why. In practice, this means they “learn through feedback” rather than through abstract information.
This approach helps to turn passive content consumption into more active learning. In the PSI model instruction happens in the context of having done and experienced something. This method of active, scenario-based learning with “instruction as reflection and feedback” is proven to be an effective strategy to achieve three key things:
Intrinsic Motivation: as an active participant in an experience that is deeply relevant to my context, I am motivated to stay, participate and learn.
Measurable Knowledge & Skills Development: as an active participant in an experience that requires me to take action and make decisions in “close to real” conditions followed by instruction via feedback, I am significantly likely to develop conceptual understanding and skills.
Learning Transfer: as an active participant in an experience that requires me to take action and make decisions in “close to real” conditions, I am much more likely to be able to transfer what I learn immediately to my day to day role.
Example: Traditional vs. Optimised Design
In practice, the shift from traditional to more innovative, evidence-based design practices looks something like this:
3. Evaluation & Iteration
The Problem: Evaluating Learning Based on Clicks
Most online learning is measured by completion rates and quiz scores, which do not indicate whether learners substantively developed any new knowledge, skills and behaviours.
This creates a situation where most online learning experiences are not just irrelevant and disengaging but also deeply ineffective, with minimal measurable impact on what learners know, what they can do and how they behave (Brinkerhoff & Montesino, 2023).
The Shift: Measuring Learning Transfer & Relevance
Instead of tracking completion rates, evaluation should focus instead on:
Competency tracking: aka, how far were concepts and skills from the training applied in real-world settings? Short Scrap Learning surveys can be a great way to achieve this in the short term.
Learner alignment: aka, collecting qualitative data on the relevance, value and impact of the training on our target learner. This data can be used to refine our personas and in turn create hyper-learner-centred experiences.
Full Comparison Table: Old vs. New Processes for Online Async Training
Overall, there are a few small but significant changes that we can make to how we design online learning in order to motivate our learners to engage and complete what we build for them, rather than delegate it to AI.
Here’s a summary:
Conclusion: The Real Threat is Not AI —It’s Poor Quality Design
The rise of agentic AI should not be viewed as a threat but as an opportunity. Agentic AI has exposed the age-old shortcomings of content-heavy, test-based courses and underscored the need for learner-driven, decision-based learning.
Our challenge and call to action to instructional designers and educators is not to create AI-proof courses, but to design learning that is so engaging and effective that humans don’t want to delegate it to AI in the first place.
Overall, my view is this: AI has not diminished the importance of learning—it has challenged us to make it better.
Happy innovating!
Phil 👋
PS: If you want to get hands-on with AI and learning science supported by me and a cohort of people like you, apply for a place on my AI & Learning Design Bootcamp.
PPS: You can download a PDF overview of my top tips for how to design online learning in the agentic AI era via Gumroad 👇