How Close is AI to Taking Over the Role of the Instructional Designer?
The Results Part 1: Writing Learning Objectives
The AI industry moves fast, which leads to lots of confusion about what AI is actually good at. AI companies are constantly testing their latest models’ performance on speed and quality, but these benchmarks don’t tell us how much of your job AI is able to potentially replace, now or in the near future—which is really what we care about.
So, last week, I kicked off an experiment to try to answer in a more structured way a question I get asked a lot: How close is AI to taking over the role of the instructional designer (ID)?
To explore this, I selected three common ID tasks of varied complexity:
Writing learning objectives.
Selecting an instructional strategy.
Creating a course outline
I then enlisted three colleagues to complete these tasks using the same course design brief:
Colleague 1: An experienced instructional designer, who completed the tasks without any AI assistance. Hypothesis: Old school methods (no AI) are best.
Colleague 2: A “novice” colleague who isn’t an instructional designer and completed the three tasks with help from AI (ChatGPT 4.0 + Consensus GPT). Hypothesis: AI enables anyone to “do an ID’s job” effectively.
Colleague 3: An experienced instructional designer who is an instructional designer and completed the three tasks with help from AI (ChatGPT 4.0 + Consensus GPT). Hypothesis: AI enhances the speed and quality of experienced IDs' work.
Next, I asked my network to blind-score the quality of the outputs for each task and try to guess which were produced by expert ID, expert ID + AI and novice + AI.
Over 200 instructional designers from around the world took me up on my invitation - with some very interesting results.
In this week’s post I will dive into the results from task 1: writing learning objectives. Stay tuned over the next two weeks to see all of the the results.
Task #1: Write Learning Objectives
One of the most common complaints I hear about AI is that it doesn’t know enough about how to properly write and sequence learning objectives to be able to do as good a job as a human ID.
This concept is so pervasive that I’ve event heard people claim that they use ChatGPT to demonstrate bad practice when teaching others how to write learning objectives.
With this in mind, the findings of the results of this first part of experiment are very interesting…
🥉 Third Place: Instructional Designer (no AI)
The learning objectives rated most poorly by respondents were those created by the human instructional designer who had no assistance from AI.
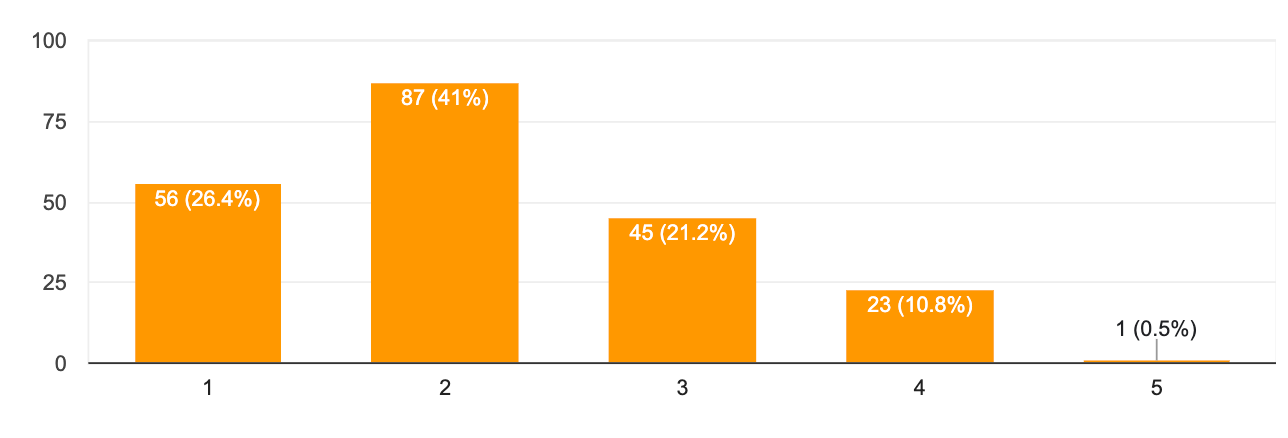
67% of respondents classified these objectives as poor or very poor. The results weren’t all bad; 21% considered the objectives to be good, and 11% very good suggesting some notable variability and inconsistency in how instructional designers define what “good” learning objectives look like.
I asked respondents to explain why they gave the scores they did - and there were some intersting themes in their responses which provide glimpses into how we define high-quality learning objectives:
Lack of Measurable & Actionable Verbs:
"None of the learning objective verbs are measurable."
"They don't have a good action verb (concrete, measurable)."
"Not measurable language like 'understand' and there are multiple targets in each objective where one might be achieved but another not."
"Doesn't follow Bloom's."
"Outcomes aren't SMART – especially not specific or measurable."
Too Wordy & Overloaded with Information:
"Too wordy, too many concepts per objective and not all can be demonstrated or measured."
"Each objective is too long."
"The objectives are too long and too full of information."
Appear More Like a List of Topics Rather Than Objectives:
"These look more like a table of contents rather than learning objectives."
"It's unclear what the learner will achieve."
"These are very info-centric course objectives that don't focus on behavioural outcomes."
"The objectives are focused on passive knowledge, not on application."
Perceived Lack of “Human Touch”:
"Feels like initial AI wrote and curated due to structure and language, but has had some human editing."
"Causality feels human. Specificity feels human. But, I kind of feel like an AI helped write and summarise."
The next question I asked was: who do you think wrote these learning objectives?
Most (64%) assumed that they had been written by a novice + AI, suggesting that the majority of instructional designers still consider high quality instructional design to be dependent on the presence of an expert, human ID.
Interestingly, 26% of respondents spotted correctly that the objectives were written by a human ID without AI, suggesting that among some there is an association between the use of AI and improved professional performance.
🥈 Second Place: Novice + AI (ChatGPT 4o)
Next, things got very juicy...
A whopping 60% of respondents considered the learning objectives created by a complete novice with some help from AI as very good or exceptional. Only 13% considered these learning objectives to be poor or very poor.
What did respondents like about these objectives?
Measurable & Actionable:
"These are well written and measurable."
"Clear, measurable and actionable."
"Good, measurable verbs used that require different levels of learning to achieve."
"These objectives use much stronger verbs (Analyse, Design, Develop...)."
"LOs are clear and concise and demonstrate use of Bloom's revised taxonomy."
Perceived “Human Touch”:
"Concrete, active verbs are used - which is typically taught to IDs when they are trained."
"They're delightfully short, possibly because the human grabbed single ideas at a time to focus on while reading the syllabus and writing them out."
Here, we see an emerging theme in associating high quality work with specialised human expertise and low quality work with AI. In reality, the opposite is true: when it comes to writing learning objectives, the use of AI, rather than the expertise of the human using it, is the biggest indicator or quality.
The next question I asked was: who do you think wrote these learning objectives?
The majority of respondents assumed that they had been written by an expert ID, either on their own (35%) or with AI (44%).
This score suggests that while “real” ID expertise is considered to be critical to producing quality learning objectives, there is an appreciation among some (specifically, 44% of respondents) that an expert ID + AI can generate better results than an expert ID on their own.
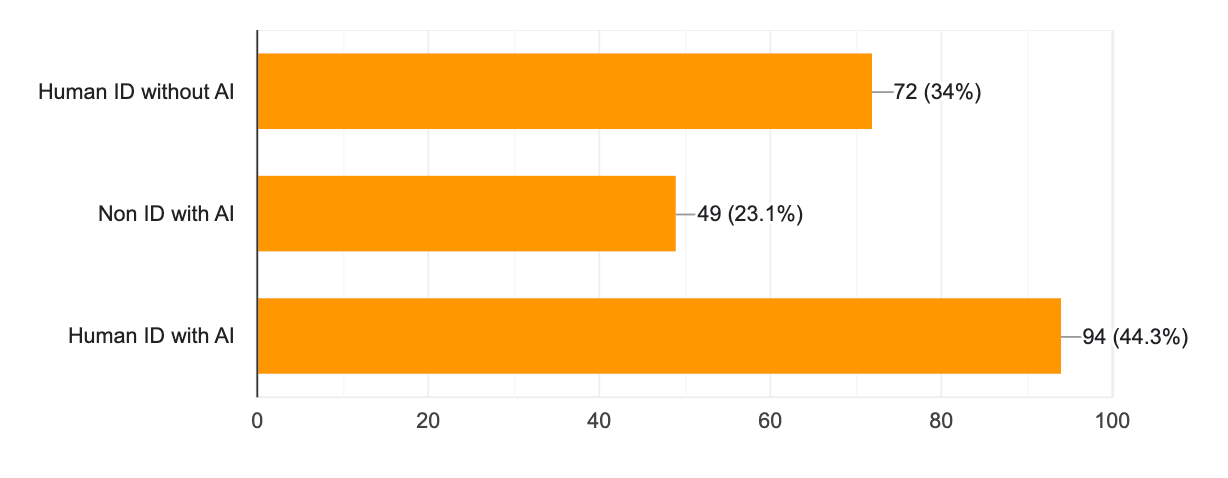
🥇 First Place Place: Instructional Designer + AI (ChatGPT 4o)
The prize for best learning objectives goes to… the instructional designer helped by AI. A whopping 71% of respondents rated theses learning objectives as good or very good. 30% of respondents rated them as exceptional. This is almost 3X the score of learning objectives written by an ID without any help from AI.
22% of respondents rated the objectives as “good”, and 7% as poor or very poor, suggesting again some variability and inconsistency in how instructional designers define quality learning objectives.
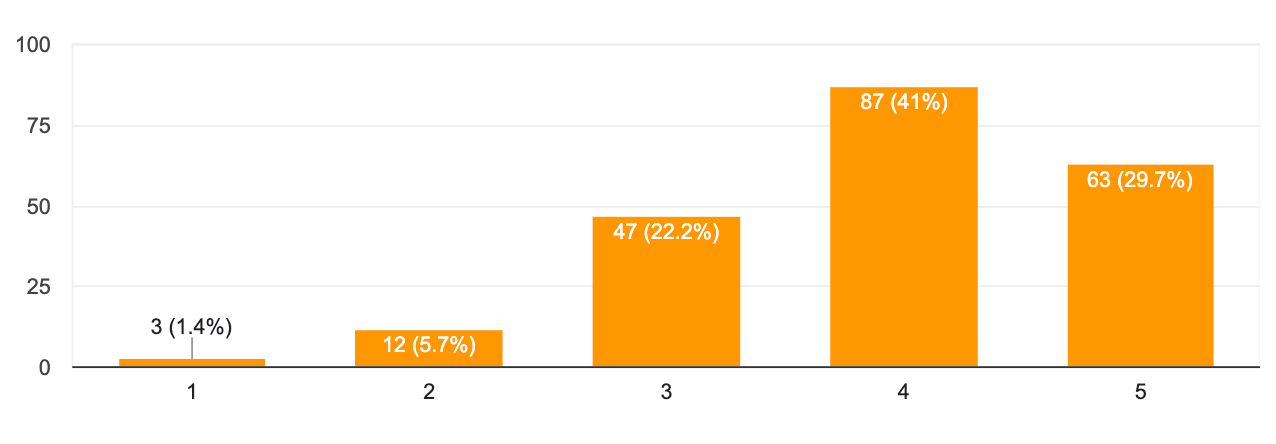
What did instructional designers like about these objectives? Again, some intersting themes emerge from their comments:
Measurable & Specific:
"Very clear actionable statements that explain what a student will do."
"Linked closely to activities, and therefore properly SMART – especially time-bound, measurable outcomes."
"Good detail, broad scope. Answers appear to follow a pre-defined template that an AI may have been told to use as result structures.
Structured & Sequenced:
"Structure is consistent, includes benefits to learner."
"This one feels the most cohesive and offers a good explanation of why the skillset is important to the bigger marketing plan picture."
"This is clear and ordered in the way a curriculum would be from beginning to end."
Learner Centred:
"Because the learner-centered approach ('you will') was added, it makes me think a human added that."
"Love how this is written in very clear and inviting language."
"This shows much more awareness of the learner and indicates clearly what they will do or achieve. It 'feels' more personal."
The next question I asked, of course, was: who do you think wrote these learning objectives?
The majority of respondents (50%) correctly assumed that high quality learning objectives were the product of an instructional designer supported by A, suggesting that some IDs acknowledge the potential of AI to enhance the ID’s performance when it is used by an expert.
At the same time, 37% of respondents associated high quality with human expertise and the absence of AI.
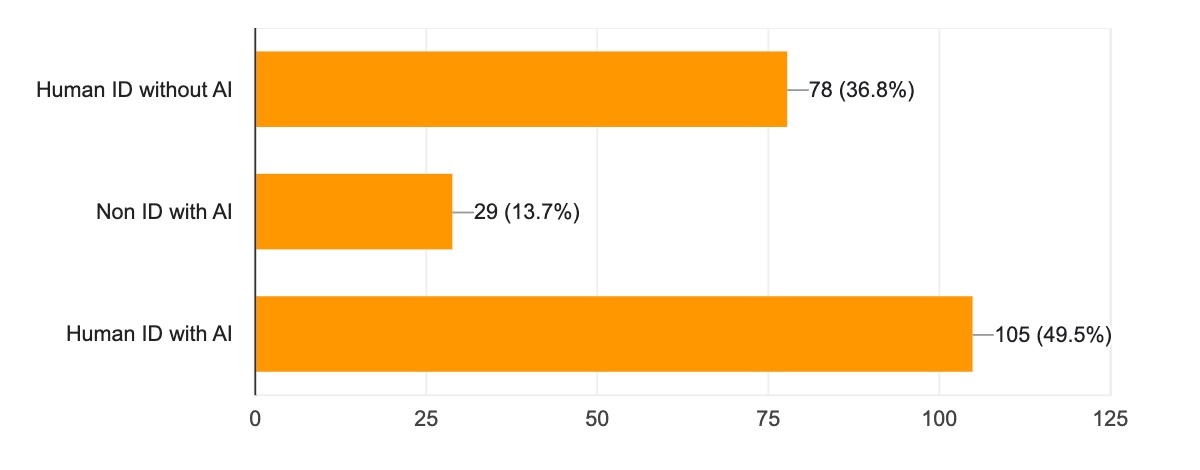
Concluding Observations
So what can we learn from this first test?
Association of quality with human expertise: Many respondents assumed that high-quality outputs were produced by human experts without AI assistance, revealing a bias towards human expertise.
Emerging recognition of AI's potential: A significant proportion of respondents (~50%) recognised that AI could enhance an expert ID's work, indicating a growing awareness of AI's potential in the field.
Misconceptions about AI-generated work: Respondents often misattributed AI-assisted work to human experts, suggesting that AI's capabilities exceed many IDs' expectations.
Variability in quality assessment: There was notable inconsistency in how IDs defined and recognised high-quality learning objectives, regardless of whether AI was involved.
The role of prompting: unstructured, conversational prompts tended to result in poorer quality outputs than structured prompting frameworks like CIDI (context, instruction, details and input).
Where can AI can help IDs most?
Writing measurable & actionable learning objectives: AI-assisted objectives were consistently rated as more measurable, specific, and aligned with best practices like Bloom's Taxonomy.
Improving consistency & structure: AI-generated objectives were noted for their consistent structure and sequencing, suggesting AI can help standardise quality.
Enhancing novice performance: The strong performance of the novice + AI combination indicates that AI tools can significantly boost the capabilities of those with limited ID experience. This aligns with research by Harvard and Boston Consulting Group, which found that AI had most significant positive impact on both efficiency and effectiveness when used by novices.
Increasing efficiency: The high quality of AI-assisted work suggests that AI can help IDs produce better results more quickly.
Overall, while there's still some skepticism and misconceptions about the role of AI in instructional design, the research suggests that AI has significant potential to enhance ID work, particularly in structured tasks like writing learning objectives.
Subscribe and stay tuned for the results from the next two parts of the research over the next two weeks.
Happy experimenting!
Phil 👋
PS: If you want to get hands on and experiment with AI supported by me, you can apply for a place on my AI-Powered Learning Design Bootcamp.