AI-Powered Learning Design: Mapping the Jagged Frontier
Practical strategies for leveraging AI’s strengths & avoiding its weaknesses
Last year, Harvard published a paper titled "Navigating the Jagged Technological Frontier" - a paper explored the impact of AI on the speed and quality of everyday work tasks. The study identified two things:
AI - specifically, Poe, Claude, and ChatGPT - can significantly enhance the speed at which we work and the quality of what we produce, IF those tasks are within its capabilities (aka - tasks within the AI frontier).
If tasks sit outside of AI’s current capabilities, its use has a negative impact on both productivity and quality (aka - tasks outside the AI frontier).
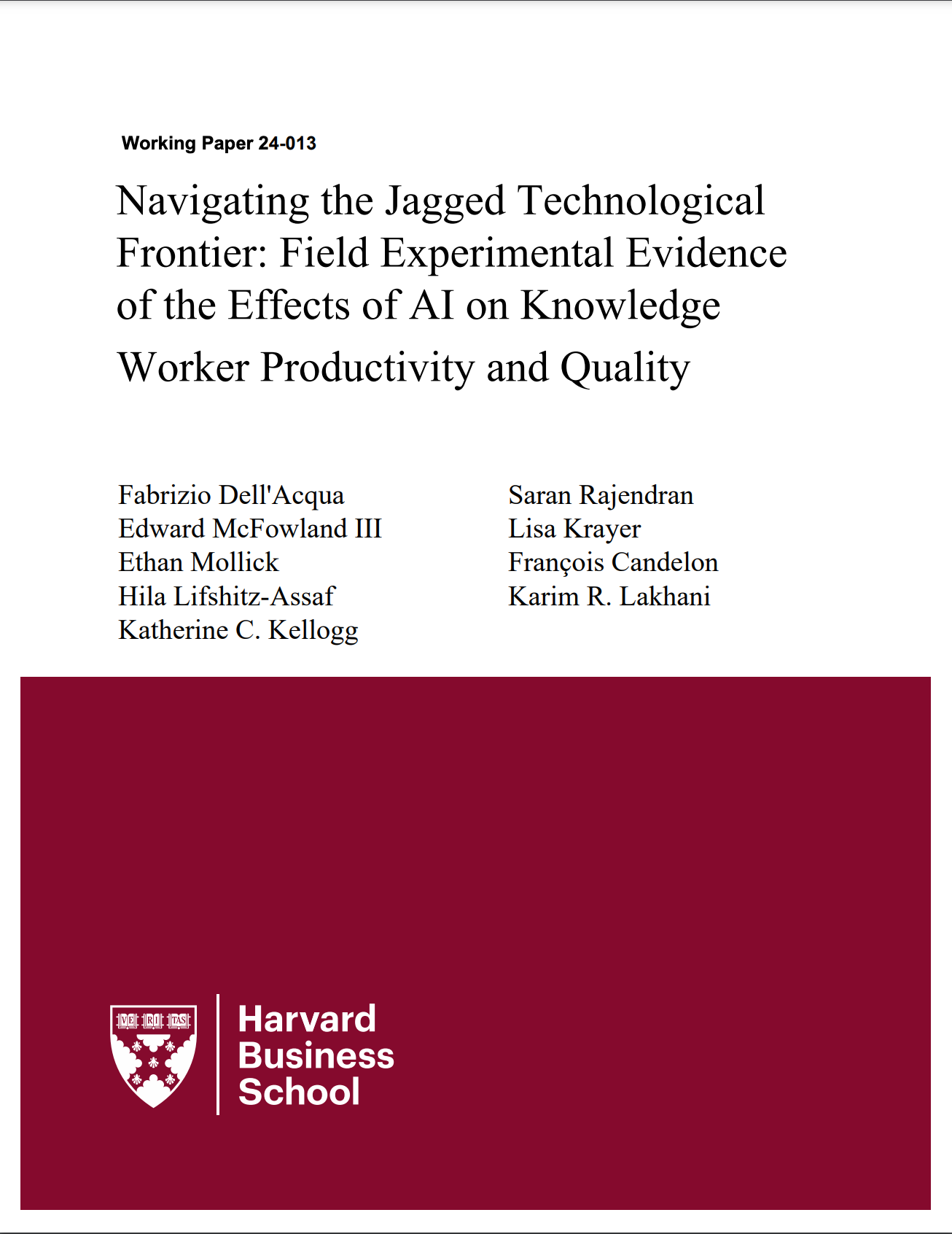
Here's a summary of the paper’s key findings:
Tasks Inside of the AI Frontier:
Productivity Booster: For tasks that fall within the AI's capabilities, the use of AI led to a significant increase in productivity. Consultants using AI were able to complete 12.2% more tasks on average and completed tasks 25.1% more quickly.
Quality Enhancer: The quality of work produced by consultants using AI for tasks within the frontier was over 40% higher compared to those not using AI.
Benefit Across Skill Levels: Consultants across the skill distribution, especially those below the average performance threshold, saw substantial benefits from AI augmentation.
Tasks Outside of the AI Frontier:
Decrease in Performance: For tasks chosen to be outside the AI's capabilities, consultants using AI were 19 percentage points less likely to produce correct solutions compared to those not using AI.
Risk of Misapplication: The use of AI for tasks it's not suited for can lead to decreased performance, highlighting the importance of understanding the limits of AI's capabilities.
Overall, the study emphasises some of the complexities which come with working with AI. TL;DR: working with AI can both significantly enhance but also potentially degrade the quality and speed of our work, depending on the nature of the task and the nature of AI's capabilities.
Defining the Jagged AI Frontier
For learning designers, Harvard’s research underscores the importance of strategically integrating AI into our workflows, leveraging it for specific tasks which sit “inside the frontier” and avoiding tasks which sit “outside of the frontier”.
Inside the AI Frontier
Tasks inside the frontier are those that AI is currently capable of performing effectively. The reason AI can perform these tasks effectively is due to the fact that they are highly structured both in terms of the instructions to complete the task and the outputs it produces. This structure means that AI can rely on its super-powers of pattern recognition and rule-based processing to produce results of a higher quality than a human can produce alone, at a faster pace.
These tasks typically involve processing large volumes of data, executing predefined procedures, or generating content based on existing templates or examples. For example, analysing data sets to identify patterns or trends and generating routine reports or summaries based on structured data are tasks that fall within the AI's capabilities.
Outside the AI Frontier
On the other hand, tasks which fall outside the frontier are those which AI struggles with due to their complexity and/or the fact that they require specialised domain knowledge and understanding.
These tasks typically involve making nuanced decisions, drawing on a large volume of varied data and drawing on specialist domain expertise which sit beyond AI’s “generalist” capabilities.
For example, when AI was asked to interpret and provide a strategic recommendation using a range of financial data and insights, it didn’t perform well. Similarly, when asked to analyse a company’s performance and identify issues and opportunities for revenue growth across specific brands, a lack of specialised understanding meant that AI did not perform well and made the task more time consuming.
Navigating the Jagged AI Frontier in Learning Design
The big questions for us, of course, is: which day to day learning design tasks sit within AI’s current frontier, and which sit outside of the frontier? As learning designers, where should we work with AI and were should we go it alone?
Inside the AI-Powered Learning Design Frontier
Many of the day-to-day tasks completed by learning designers are structured both in terms of the instructions we can give to complete the task and the outputs the task produced produces. For these tasks, AI has the potential to boost speed by ~25% and quality by ~40%.
Here are some tasks to try out, mapped to the ADDIE process:
Analysis > Survey and Feedback Analysis
AI can process large volumes of “single type” survey data or learner feedback to identify common themes, sentiments, and patterns. This is possible because AI excels at text analysis and natural language processing, enabling it to quickly synthesise feedback from learners or stakeholders to highlight areas of need or interest.
Design > Content Curation
AI can assist in curating existing content from large databases or the internet based on specific learning objectives. This task sits inside the frontier because AI can efficiently sift through vast amounts of data to find relevant information, using simple keyword matching and semantic analysis.
Development > Generating Learning Content
AI can generate structured content like summaries, definitions, slide decks and quiz questions based on clear instructions and existing example and templates. This task draws on AI's ability to learn and repeat predefined patterns and rules to produce coherent, context-appropriate content.
Implementation > LMS Management
AI can assist in automating structured LMS-related tasks like learner enrolment and the analysis of learner data. The first task leans into AI’s strengths in that it’s highly structured both in terms of the instructions to complete the task and the outputs it produces. The second task draws on AI’s ability to rapidly complete structured tasks text analysis and natural language processing, enabling it to quickly synthesise data and highlight areas of need or interest.
Evaluation > Analysing Learning Performance
AI can process and analyse test scores and other performance data to identify trends and patterns in learner achievement. This task falls within AI's capabilities of handling large data sets and executing complex statistical analyses more quickly and accurately than manual methods.
These tasks align with AI's strengths in processing structured data, recognising patterns, generating content based on templates, and applying predefined procedures. By leveraging AI for these tasks, learning designers can focus more on specialist complex, creative, and strategic aspects of instructional design that require human insight and expertise.
Outside the AI-Powered Learning Design Frontier
Tasks which fall outside the frontier are those which AI struggles with due to their complexity and/or the fact that they require specialised domain knowledge and understanding.
These tasks typically involve making nuanced decisions, drawing on a large volume of varied data and drawing on specialist domain expertise which sit beyond AI’s “generalist” capabilities.
Here are some learning design tasks which - for now at least - sit outside of the jagged AI frontier:
Analysis > Problem Identification
Although AI can process data to identify patterns, developing a deep and contextualised understanding of the problem we’re trying to solve for a specific business and/or learner is beyond the capability of most LLMs.
Design > Evidence-Based Design Decisions
AI’s “generalist” training means that it lacks the domain expertise and understanding to make robust decisions about instructional and assessment strategies based on our goals and target learners.
Development > Instructional Scripting
Although AI can rapidly produce video, audio, quizzes etc, it does not have the specialised domain knowledge to ensure that the scripting of those elements is optimised for learner motivation, engagement and impact. For this, it relies on the depends of the human learning designer.
Implementation > Facilitation
Although we speak a lot about AI coaches and instructors, without specialised AI expertise and complex model development, AI is not yet capable of facilitating learning in a way that is guaranteed to support each student consistently and fairly. For this, we still rely on human facilitation.
Evaluation > Measuring Impact
Determining the effectiveness of a learning experience requires us to combine large quantities of both qualitative and quantitative data, including learner performance data, qualitative feedback and “on the job” observation data. This comprehensive evaluation, combining multiple sources of qualitative and qualitative data with pedagogical insight and defining interventions tailored to a specific context and goal, is beyond AI's current capabilities. For now at least, robust learning evaluation and evaluation-based iteration demands a level of nuance and complexity which is best handled by a human.
Conclusion
"Navigating the Jagged Technological Frontier" offers some compelling insights into the integration of AI within the field of learning design. The research highlights a complexity and nuance around working with AI isn’t often acknowledged - i.e. that integrating AI into our day to work is neither a good nor a bad idea; it’s something which can both increase the speed and quality of our work and - at the same time - slow us down and reduce our effectiveness.
Perhaps the key take away from this research is that AI Needs You. We’re often told that AI needs us to prompt it well and analyse its outputs. But more fundamentally, AI also needs you to decide when to use it, and when to work solo.
Here are some practical tips to help you navigate this jagged frontier:
Embrace AI for Structured Tasks: As learning designers, we should actively incorporate AI tools for tasks that are highly structured and data-driven, such as survey analysis, content curation and basic performance analysis. These tasks benefit from AI's ability to process large volumes of data and identify patterns, significantly reducing time spent on these activities and allowing designers to focus on more complex aspects of learning design.
Understand the Limits of AI: Recognise the boundaries of AI's capabilities, especially in tasks requiring deep domain expertise, nuanced decision-making, and the integration of varied data sources. For tasks like problem identification, evidence-based design decisions, and measuring the impact of learning interventions, human expertise remains irreplaceable. Learning designers should critically evaluate where AI can be beneficial and where its limitations might impact the quality of learning outcomes.
Augment Human Expertise with AI: Use AI as a tool to augment, not replace, human expertise in learning design. For instance, while AI can generate learning content rapidly, human designers must refine this content to ensure it aligns with learning objectives and engages learners effectively. This approach leverages the best of both worlds—AI's efficiency and the depth of human understanding.
Learn How to Test & Integrate AI Tools: As AI continues to evolve, learning designers must cultivate the skills to effectively integrate AI tools into their workflow. This includes understanding the capabilities of different AI technologies, learning to customise AI-generated content, and staying informed about advances in AI that could impact learning design.
Develop Skills Which Sit Outside of the Frontier: To stay relevant in a post-AI world, focus on developing skills that complements, rather than competes with, AI”s capabilities. This includes developing deep knowledge of learning theories, instructional design principles, and the science of how people learn.
The strategic integration of AI in learning design offers immense potential to enhance the efficiency and quality of the work of learning designers and, bigger picture, the quality and impact of learning experiences.
By strategically applying AI to tasks within its capabilities and recognising the unique value of human expertise, learning designers can optimise their workflows and use AI to contribute to the creation of more effective and engaging learning experiences. As we navigate the jagged technological frontier, the synergy between human insight and AI's power will be instrumental in shaping the future of education.
Happy innovating!
Phil 👋
If you want to learn more and get hands-on with AI, you can apply for a place on my AI Learning Design Bootcamp.