Hey folks!
This week on my AI & Learning Design Bootcamp, we wrapped up our exploration of AI’s impact on each stage of the ADDIE model with Evaluation—arguably the most overlooked phase of instructional design.
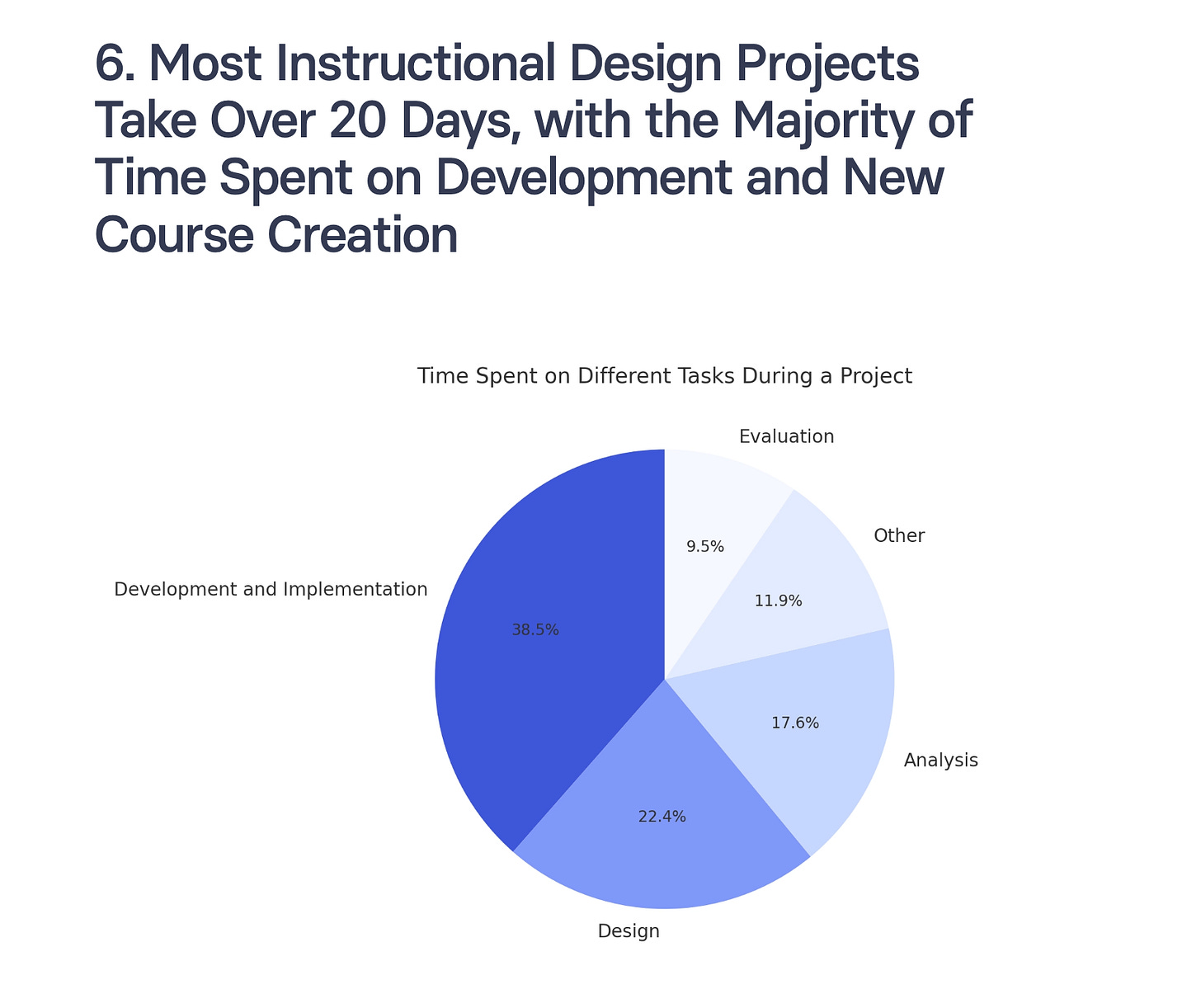
Over the past three weeks, we have explored if and how AI can enhance Analysis, Design, Development and Implementation. This week, we turned our focus to if and how AI can make the evaluation part of process not just faster but also more effective.
Through hands-on experiments, we found that AI has the potential both the accelerate and augment the evaluation part of ADDIE. Specifically, we found four areas where existing AI tools can make us both faster and better at evaluation:
Designing Evaluations – AI can help instructional designers determine what to measure, which methods to use, and how to structure evaluation questions to gather meaningful data.
Executing Evaluations – AI can automate and scale data collection, using chatbots, survey tools, and learner simulations to gather richer and more reliable insights.
Analysing Evaluation Data – AI can process large volumes of both quantitative (e.g., usage stats, feedback scores) and qualitative (e.g., open-ended responses, discussions, interviews) evaluation data, extracting trends and insights faster than manual analysis.
Implementing Evaluation Data – AI can recommend course improvements based on evaluation findings and even predict whether proposed design changes will lead to improved learner outcomes.
In this post, I share the use cases we explored in the bootcamp and give you the info you need to try them for yourself.
Let’s go! 🚀
Step 1: Working with AI to Design Evaluations
Use Case 1: Find the Best Evaluation Method
💡 Why? Choosing the right evaluation method (Kirkpatrick, ROI, Success Case Method, etc.) can be overwhelming.
🛠 Try This:
Use Consensus, DeepSeek, Perplexity Deep Research or STORM by Stanford to compare evaluation methods.
Example prompt: “What are the best evaluation methods for measuring X behavioural changes in leadership training for [learner type & context, e.g. in banking]?”
AI will provide research-backed methods—pick the one that aligns with your objectives.
Use Case 2: AI-Generated Evaluation Questions
💡 Why? The quality of your questions impacts the quality of your evaluation data.
🛠 Try This:
Use ChatGPT, Claude, or Co-Pilot to create targeted evaluation questions.
To get started, copy-paste this prompt: “Generate the minimal viable number of evaluation questions for a cybersecurity training program that measure learners’ ability to apply security protocols in real-world scenarios.”
Want to take a more structured approach? Try this prompt: “Write evaluation questions for a [course type] for [target learners] which aims to achieve [intended impact] based on Kirkpatrick’s four levels of evaluation.”
Step 2: Working with AI to Execute Evaluation
Use Case 1: AI-Powered Evaluation Bots
💡 Why? AI chatbots can collect richer, deeper post-training insights than traditional surveys.
🛠 Try This:
Use ChatGPT GPT Builder, Poe Bot Builder, Poe App Builder, or Replit Agent to create a chatbot that conducts post-training interviews.
Train it to ask follow-up questions for vague responses.
Example:
Learner: “The training was useful.”
AI: “Can you provide a specific example of how you applied what you learned?”
Use Case 2: Predict Learner Feedback Before Launch
💡 Why? AI can predict potential learner responses before you even roll out your course.
🛠 Try This:
Use ChatGPT 4o to simulate learner feedback. This can be quite complex, but if you get it right it produce unprecedented gains in both speed and quality.
Read this article to understand how to build a persona, then work with ChatGPT 4o to test if and how well it can simulate your learners. Tip: start by positioning yourself as the learner to test how well it predicts your needs.
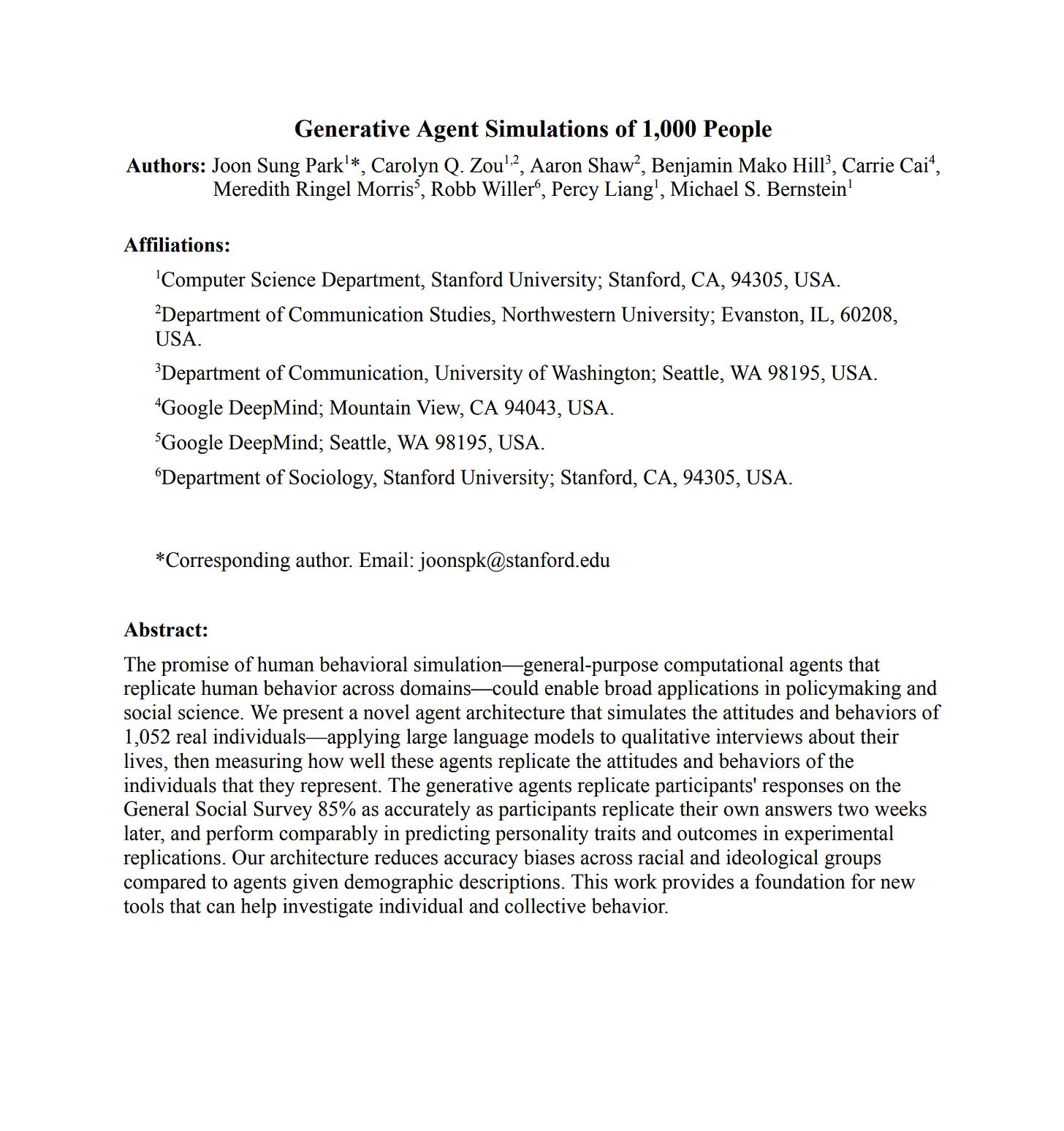
Step 3: Work with AI to Analyse Evaluation Data
Use Case 1: AI for Quantitative Data Analysis
💡 Why? Certain AI models can quickly process survey responses, engagement metrics, and assessment scores.
🛠 Try This:
Grab existing quantitative data from feedback, LMSs etc and use a free trial of Julius AI to identify trends in:
Learner engagement
Completion rates
Assessment scores
Use Case 2: AI for Qualitative Data Analysis
💡 Why? Some AI models are pretty good at semantic analysis - i.e. they can analyse text-based data like open-ended responses, discussions and evaluation interviews.
🛠 Try This:
Use Consensus, Perplexity or STORM to understand how to run meaningful semantic analyses on commonly generated text-based data like open-ended responses, discussions and evaluation interviews
Use ChatGPT, Claude, or Co-Pilot to analyse qualitative data from:
Slack & Teams channels
Post-training discussion forums
Learner interviews
Use Case 3: AI for ROI Calculation
💡 Why? Measuring Return on Investment (RoI) is one of the most wicked problems faced by instructional designers. With the right data to hand, AI can help measure the return on investment (ROI) of training programs by analysing cost/impact using complex calculations.
🛠 Try This:
Step 1: Collecting Data
To calculate ROI, gather:
Training Costs: Development, materials, delivery, participant time costs.
Performance Data: Productivity, quality, cost reductions, revenue increases.
💡 Beginner Tip: Use AI to generate dummy data and practice your ROI calculations.
Step 2: Input Data & Analyse ROI
Use ChatGPT, Claude, Julius AI, or Co-Pilot for calculations.
Try this prompt: “I have collected the following training cost data: [list of costs]. The performance data before training was [X], and after training it was [Y]. Can you help me calculate the ROI using the Phillips methodology?”
Step 3: Isolating Training Effects
Use AI for trend analysis, comparing performance trends before and after training.
Copy-paste this prompt: “Here is performance data before and after training: [data]. Can you help me apply trend line analysis to see if training was the cause of improvement?”
Step 4: AI-Powered Visualisation & Reporting
Use Julius AI, ChatGPT-4o, Claude, or Vo by Vercel to create interactive graphs and dashboards.
Copy-paste this prompt: “Using the data attached, generate an interactive bar chart comparing pre-training and post-training performance.”
Step 4: Working with AI to Implement Evaluation Data
Use Case 1: AI-Powered Course Redesign
💡 Why? AI can analyse feedback and suggest data-driven improvements.
🛠 Try This:
Use ChatGPT, Claude, Co-Pilot, or Julius AI to analyse learner feedback and suggest improvements to:
Learning objectives
Course content
Activities
Delivery modes
Course length
Copy-paste this prompt: “Based on this evaluation data, what improvements should be made to course content, delivery, and structure, and why?”
Use Case 2: Rapid Prototyping
💡 Why? AI can enable us to rapidly create prototypes of updated designs to test with learners.
🛠 Try This:
Use Vo Vercel to rapidly create a prototype of the updated course designs.
Use ChatGPT, Claude or Co-Pilot to rapidly create a set of questions to ask learners about their experience in order to assess the impact of the updated course.
Use the data to make final changes and improvements to the design.
Final Thoughts
Getting hands on and trying out AI tools with fellow learning professionals in the bootcamp has reinforced two things:
1. AI is not just a tool for automation—used correctly, it’s a thinking partner that helps instructional designers take a more strategic, evidence-based approach to learning measurement.
2. AI needs you (and you need instructional design expertise)—in order to get the most from AI, you need to understand what to ask it to do, and how to assess the quality of its output.
TLDR: in the age of AI, your domain expertise as an instructional designer is arguably more important and more powerful than ever.
Happy innovating!
Phil 👋
PS: If you want to get hands-on with AI supported by me and a cohort of people like you, apply for a place on my bootcamp.