AI in Instructional Design: reflections on 2024 & predictions for 2025
Aka, four new year's resolutions for the AI-savvy instructional designer.
I've spent some time in the last week or so reflecting on how instructional design roles have evolved this year—and how they will likely change again in 2025.
As the year comes to a close, here's my review of how the world changed for instructional designers in 2024, my predictions for what's to come in 2025 (and beyond) and what this means for you, your role, key knowledge and skills.
Spoiler - my hypothesis is that instructional design is moving through three stages of AI mastery:
2024: The Year of AI Experimentation - Most Instructional Designers started to explore using AI as a tool to help with basic everyday tasks like brainstorming and content summarisation.
2025: The Year of Prompt Engineering - Instructional Design roles will increasingly require specialised expertise in prompt engineering with the goal of turning experimentation and potential into impact. In turn, some Instructional Designers will hone both their domain and AI expertise to meet this demand.
2026: The Year of Reward Engineering - The most innovative Instructional Designers will evolve from AI users to AI builders, partnering with engineers to train specialised language models for education / instructional design.
Let's dive in!
2024: The Year of AI Experimentation (But Not Impact)
One thing is clear: 2024 was the year that instructional designers adopted AI. As various reports published this year have shown, the adoption of AI among instructional designers has been both rapid and widespread.
According to surveys like the Learning at Work research conducted by the CIPD in 2023, rates of experimentation with AI among learning professionals was very low, with only 5% of respondents declaring they had tried the tool in their work. Even if allowing for the existence of so-called “Secret Cyborgs” which we discussed later in the year, in 2023 the number of learning professionals using AI was notably low.
In 2024, the picture was very different.
In a global survey I ran with Synthesia in October 2024, for example, we found that:
84% of instructional designers have used ChatGPT in their work.
49% of instructional designers use AI in their daily work.
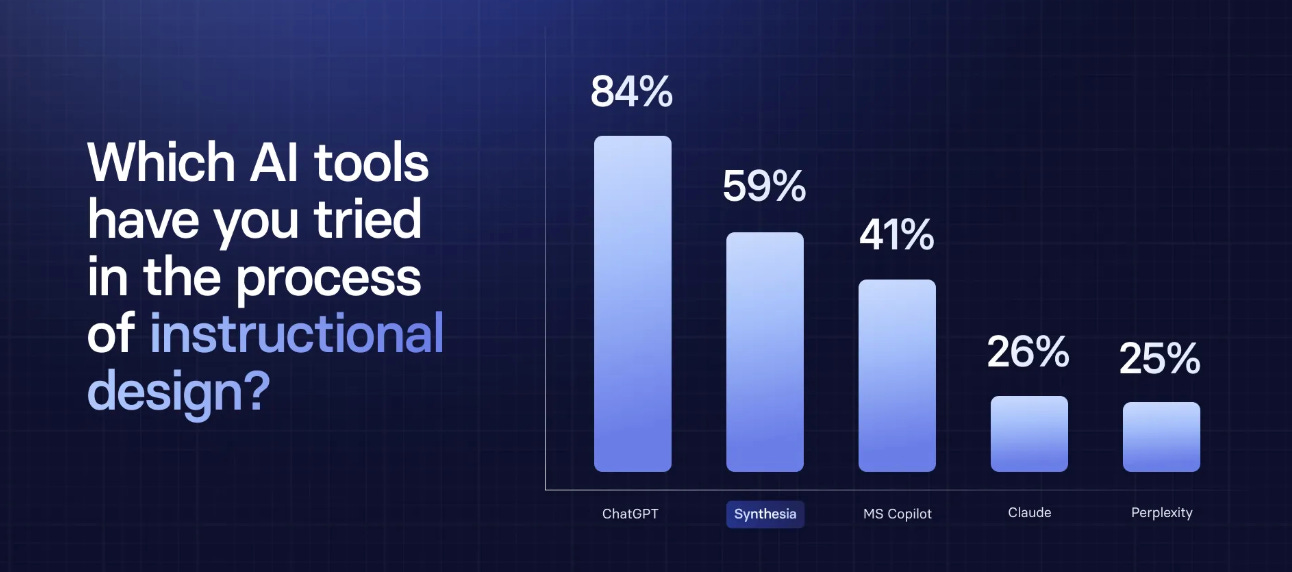
As Taylor and Vinauskaite reported in their 2024 survey of the state of AI in L&D, “If 2023 was the year of L&D’s fascination with AI, then 2024 is the year of action.”
The Impact of the Increasing Use of AI
The some-might-say stratospheric rise in the use of AI among learning professionals in 2024 inevitably had some impact on how we worked. Here’s the TLDR of what changed, based on interviews I’ve run with ~200 instructional designers.
Analysis
Pre 2024: IDs manually wrote surveys, analysed and compiled survey responses and identified patterns and insights using Excel or similar tools. In reality, IDs typically had limited analytical skills and limited time for analysis, which meant that analysis was limited to basic surveying and/or (as one ID put it) “best guesses and assumptions”.
2024: AI was used to write survey questions, summarise survey responses, run sentiment analysis on learner feedback, analyse LMS data and identify skills gaps from performance data. IDs used tools like Claude or ChatGPT to quickly extract patterns and insights, which were used to inform course design decisions.
Design:
Pre 2024: IDs manually conducted domain research, created knowledge maps, wrote objectives, selected instructional strategies from academic sources, and developed course outlines. In reality, there was little time for any of these tasks, and many were skipped.
2024: AI assisted IDs with with domain research, objective writing, instructional strategy selection, and course outlining. IDs used Claude or ChatGPT to create knowledge maps, draft learning objectives, select evidence-based strategies, and build course outlines - then validated and refined these outputs with stakeholders.
Development:
Pre 2024: IDs manually wrote scripts, created multimedia content, and built course prototypes using traditional authoring tools. This included writing quiz questions, creating visuals, and recording audio/video content.
2024: IDs used AI to generate scripts, create multimedia (using tools like Synthesia for video, Ideogram for images and ElevenLabs for audio), and rapidly prototype course modules. They combined AI outputs with authoring tools to build and iterate designs before they were published.
Implementation:
Pre 2024: IDs manually handled course rollout tasks - writing welcome messages, verifying enrollment, creating onboarding guides, sending communications, providing technical support, and monitoring early engagement.
2024: IDs used AI to help write course communications, onboarding guides and other materials. Ambitious IDs experimented with building AI bots to provide 24/7 learner support, but few used them in practice.
Evaluate:
Pre 2024: IDs manually wrote and analysed post-course surveys and manually analysed data like learner participation and performance.
2024: IDs used AI to help write evaluation surveys and analyse qualitative and qualitative survey results and other data (with mixed reviews).
TLDR: if one thing is clear it’s that 2024 was the year that instructional designers adopted AI. But this is only part of the story…
What DIDN’T Change in 2024 (and Why)?
Despite widespread experimentation and adoption of AI, overall, 2024 was a year of continuity rather than change for Instructional Design.
As this global survey showed, for example, despite the widespread use of AI, a number of long term industry averages remain unchanged at the end of 2024:
96% of instructional designers who use AI still work on only 2-4 projects at once;
The majority of those who use AI still turn down a significant number of projects due to capacity constraints;
Almost one half of all IDs’ time is still spent on practical development & implementation tasks.
Why is this? The short answer is: generic AI models.
Generic AI models like those which power ChatGPT and Claude are built to behave like generalists - they’re optimised to be OK at everything, rather than exceptional at any one specific type of task.
What this means in practice is that the tools we're predominantly using in the instructional design process are not optimised for instructional design. You can read more about just exactly how bad generic AI models are at instructional design here.
The result is that without a user who can bring a) significant expertise in optimal instructional design practices and b) understanding of how LLMs and how to work best with them (aka advanced prompt engineering skills), the value we can get from generic AI models and tools like ChatGPT and Claude is severely limited.
This, paired with a lack of strategic direction and substantive AI training, explains why in 2024 we saw widespread adoption without any substantive positive impact on the speed, volume or quality of instructional designers’ work.
So, will this situation change in 2025? I think the answer is yes.
2025: The Year of the Instructional Design Prompt Engineer
As we enter 2025, a major shift already seems to be underway: the formalisation of prompt engineering as a core skill for knowledge workers in general and instructional designers in particular.
Prompt engineering refers to the skill of understanding LLMs enough to be able to craft instructions in a way that enables generic AI models like ChatGPT and Claude to produce high-quality, high-value outputs.
There are two key differences between conversational, unstructured prompts and structured or engineered prompts:
Conversational, unstructured prompts simply ask AI to execute a task using natural language structures, e.g.
Write three SMART learning objectives for an online, async workplace safety course.
Structured or engineered prompts do two things differently:
First, they ramp up both quality and reliability by telling AI not just WHAT to do, but also HOW to do it.
Second, they ramp up quality and reliability further by structuring inputs in line with how Large Language Models (LLMs) work.
Here’s an example:
Context: You are an instructional designer creating a compliance course for factory workers.
Task: Write three learning objectives aligned with your learners' ZPD and OSHA standards. You must write the objectives using the instructions below.
Instructions:
1. The objectives must be SMART:
Specific: The objective should be clear and precise, so that it's easy to understand what needs to be done and who's responsible.
Measurable: The objective should include a way to quantify progress.
Achievable: The objective should be challenging but realistic, so that it's motivating without being too stressful.
Relevant: The objective should be connected to the job role and contribute to the organisation's success.
Time-bound: The objective should include a timeline for expected results.
2. Level: The objectives must use Bloom’s Taxonomy at the “apply” level.
3. Alignment: The objectives must align with OSHA guidelines. They must also align with the ZPD of the learner profile provided and help to mitigate the risk of repetition of the incidents listed in the report provided.
Output: A list of learning objectives and a rationale which explains how they are aligned with your learner's ZPD, mapped to OSHA guidelines and optimised to mitigate the repetition of the incidents listed in the report provided.
Input: [learner profile, OSHA guidelines and recent incident report].
The result? Recent research like that by Sahoo et al., 2024; Amatriain, 2024 and Gu et al., 2023) definitively shows that when we learn how to engineer and structure prompts, we deliver consistently more reliable, higher-value outputs.
Why Will Prompt Engineering Become a Key Skill for Instructional Designers in 2025?
There are two key trends here which are converging to create the perfect storm for an explosion in demand for AI skills:
Economic Incentives: Early studies show that knowledge workers who are skilled in prompt engineering complete projects up to 40% faster and of a better quality than those using no AI or unstructured prompting.
360-Degree Demand: 79% of workers believe AI skills will broaden their job opportunities, and 76% want to develop AI skills to remain competitive in the job market. At the same time, 89% of business leaders see AI as the #1 tool for growing revenue, improving operational efficiencies and boosting customer experiences.
As a result, experts predict that the prompt engineering market will grow at 32.8% CAGR from 2024-2030, driving demand for AI-skilled professionals in all industries, including our own.
In many industries, prompt engineering is already emerging as an in-demand skill. In a range of sectors from Product Management to Copywriting and Manufacturing, we are already witnessing the emergence of dedicated roles which require not just expertise in the related domain but also the ability to work effectively with LLMs.
Interestingly, the education sector doesn't seem to be too far behind. An analysis of job descriptions at the end of 2024, shows that some education and instructional design roles already require advanced prompt engineering skills.
GT.School for example, recently advertised for a candidate who could, "Design, develop, and refine prompts that to produce high-impact educational content and build-out our core data models".
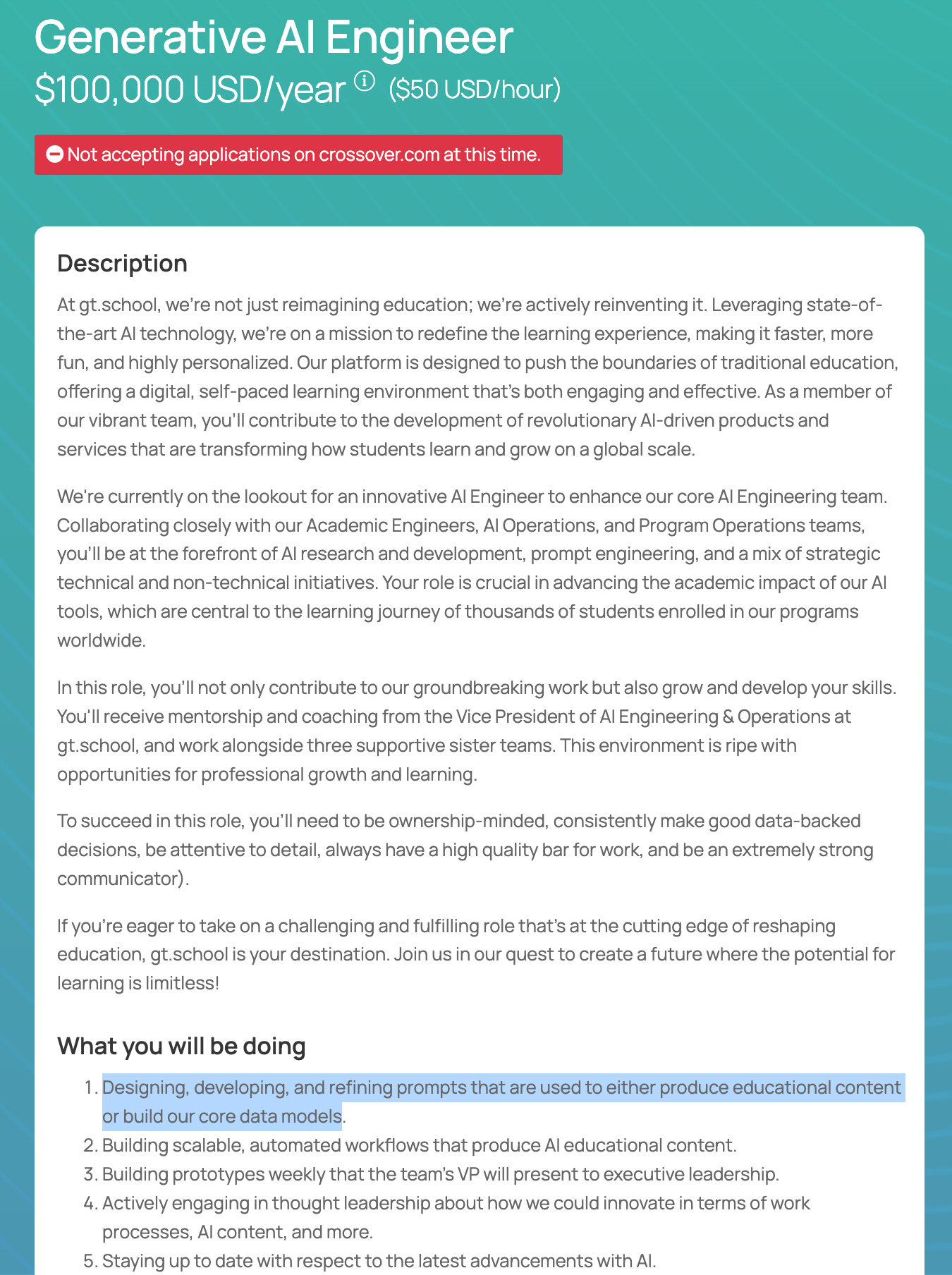
Similarly, this open role at education company SkillCat requires, “The ability to fine-tune prompts for educational purposes, with skills in adjusting prompts to achieve specific learning objectives and outcomes on specific LMSs.”
What Does The Rise of Prompt Engineering Mean for Instructional Designers?
A growing body of prompt engineering research (e.g.,Sahoo et al., 2024; Amatriain, 2024; Gu et al., 2023) highlights that successful collaboration with generic AI models requires expertise in two key areas:
Deep Domain Expertise
In order to work well with generic AI, Instructional Designers require a deep understanding of the domain. Only with a deep and clear understanding of how to execute tasks can we instruct or "teach" generic AI tools to produce high-value, high-impact outputs.
Think of working with generic AI models as working with an eager but very inexperienced apprentice: in order to optimise your apprentice's performance, you need to give them clear, detailed & structured instructions not just on what to do but also how to do it.
As apprentices, generic AI models also need their outputs to be checked by an expert to validate the quality and reliability of their work. In practice, this means that Instructional Designers need more than ever to go deep on the "how" of their craft - i.e. on understanding what optimal practices look like and using this expertise to write carefully engineered prompts in a way that optimises for value and impact.
Perhaps surprisingly, rather than automating and standardising our industry, the rise of generic AI tools have helped to place a new emphasis on the importance of the expert human in the loop.
By requiring us to become the teachers of generic AI models, the rise of generic AI models gives us as Instructional Designers an opportunity for some very valuable metacognition and professional development: it encourages us to step back, reflect in detail on our thought and decision-making processes and place new emphasis on the importance of deep domain expertise.
AI Expertise
As well as deep domain knowledge, in order to guide AI effectively we also need an advanced technical understanding of how AI systems work. A growing body of prompt engineering research (e.g.,Sahoo et al., 2024; Amatriain, 2024; Gu et al., 2023) shows that by understanding how LLMs work and behave, we are able to work with them in a way that can significantly increase both the speed and the quality of our work.
But what does “AI expertise” mean in practice? A lot of experts have asked this question in the last year and collectively have highlighted that successful collaboration with AI requires expertise in two key areas:
How AI Models Behave: Unlike human learners, AI models require carefully crafted inputs to deliver optimal results. In practice, this means developing a solid understanding of:
Model-specific processing: How different architectures (e.g., GPT-4, Claude, PaLM) interpret and generate outputs, including their inherent strengths and weaknesses for different tasks.
Parameter tuning: The impact of AI parameters like temperature and top-p on output variability and quality.
Context management: Awareness of context window limitations and strategies to structure prompts effectively within these constraints.
Token efficiency: Understanding how to use token usage to optimise output quality while controlling computational load and operational costs.
How to Operate AI Models: In order to consistently produce high-quality outputs, AI users need to understand and master a range of techniques. According to the research cited above, the key techniques to explore and master are:
Chain-of-thought prompting: verifying AI's reasoning process and catch faulty logic
Tree-of-thought prompting: exploring and evaluating multiple instructional approaches by comparing their pros and cons
Self-consistency prompting: comparing multiple outputs and identify potential inconsistencies
Constitutional prompting: ensuring alignment with learning science / pedagogical research
Four New Year's Resolutions for the AI-Savvy Instructional Designer
For those who want to lean into the brave new world of AI-powered Instructional Design in 2025, here are four resolutions to set you on a path to success:
Resolution 1: Deepen My Craft
I resolve to strengthen my domain expertise in Instructional Design by:
Taking time each month to reflect on and document my design process, surfacing my decision-making processes
Using tools like Consensus to keep on top of current research on effective methods, strategies etc
Challenging myself to explain my design choices as if teaching them to someone else
Resolution 2: Development a Mentor Mindset
I resolve to approach AI as a helpful apprentice by:
Developing clear, structured instructions for AI models on what to do and how to do it
Implementing a three-step quality assurance process for checking AI’s outputs: accuracy check, pedagogical alignment, and learner experience review
Documenting both successes and failures in AI collaboration to refine my guidance approach over time
Resolution 3: Level-Up My AI Technical Knowledge
I resolve to enhance my understanding of AI systems by:
Comparing outputs from unstructured and structured prompts across a range of major AI models (e.g. GPT-4, Claude 3.5, Llama 3, PaLM, Gemini) to understand variations in how they “think” and “work”
Running weekly comparisons of major AI models highlighting their unique strengths and weaknesses in relation to different ID tasks across the end to end ADDIE process
Run monthly experiments with temperature and top-p settings to find optimal configurations for various tasks and content types
Resolution 4: Master Advanced Prompting
I resolve to improve my prompt engineering skills by:
Dedicating weekly practice time to master key prompting techniques, including
Researching & experimenting with Chain-of-thought prompting to verify AI's reasoning process and catch faulty logic
Researching & experimenting with Tree-of-thought prompting to explore and evaluate multiple instructional approaches by comparing their pros and cons
Researching & experimenting with Self-consistency prompting to compare multiple outputs and identify potential inconsistencies
Researching & experimenting with Constitutional prompting to ensure alignment with learning science / pedagogical research
Experimenting with additional strategies like:
Iterative refinement: using AI outputs as inputs for follow-up prompts to catch and correct inconsistencies
Chain-of-verification: using direct quotes and citations to ground AI responses in source materials
Best-of-N comparison: running the same prompt multiple times to identify inconsistencies
External knowledge restriction: explicitly instructing AI to only use provided documents)
Uncertainty acknowledgment: explicitly allowing AI to admit when it lacks sufficient information
Conclusion: The Impact of AI on Instructional Design
2024 was the year that we saw widespread adoption of generic AI tools like ChatGPT and Claude. Yet, the unstructured experimentation that has characterised most AI use until now means that AI's impact so far has been incremental at best. Along with the majority of knowledge workers, instructional designers have begun to integrate AI into their processes, but the transformative leap in efficiency and quality that we anticipated remains - for now at least - unrealised.
By moving from unstructured experimentation to more intentional AI use, 2025 will likely be the year that Instructional Designers will start to produce higher quality outputs more consistently and efficiently. If this happens, 2025 will be the year that AI starts to change Instructional Design as an industry, shifting focus from functional to more strategic tasks and transforming the speed at which we work.
What does the forecast look like for AI and Instructional Design beyond 2025?
Trends already underway suggest that mastering prompt engineering is just the beginning of our AI journey as Instructional Designers. At the bleeding edge of AI technology, the act of engineering prompts to optimise generic, pre-trained AI models is already being superseded by a new focus on building reward systems for specialised, dynamic AI models.
Imagine a world where instead of crafting a prompt to get optimal outputs from a generic AI model, you instead work side by side with AI engineers to design reward systems which reinforce optimal Instructional Design behaviours. In this iteration, Instructional Designers gradually shifts from being a user of AI (prompt engineer) to co-creators of complex AI systems (reward engineer).
Example - a reward engineering system for an AI built to generate learning objectives:
Rewards:
+10 points for including all five SMART criteria.
+10 points for aligning with the learner’s ZPD.
+10 points for sequencing from simple to complex.
+5 points for aligning with relevant verbs from Bloom’s Taxonomy.
Penalties:
-10 points for each missing SMART criterion
-10 points for objectives misaligned with ZPD
-10 points for poor sequencing
-5 points for misused/missing Bloom's verbs
In both the near and longer term then, the future of AI and Instructional Design looks perhaps surprisingly bright.
There is, of course, a potential future where AI replaces Instructional Designers and standardises Instructional Design. But there’s an alternative and in many ways more likely potential future where AI elevates Instructional Design, positioning Instructional Designers first as power-users and later as co-creators of complex AI systems.
In both cases, AI offers us as the opportunity to redefine how learning is designed and delivered and to transform the speed, quality and impact of our work. Whether or not we take and build on this opportunity is up to us.
Happy experimenting and happy new year!
Phil 👋
PS: If you want to get hands-on, hone your instructional design knowledge and learn how to get the most out of AI with me, apply for a place on my AI & Learning Design Bootcamp.